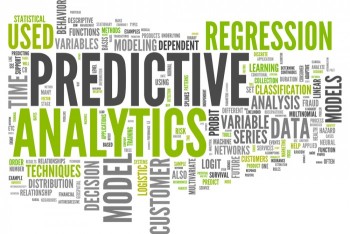
After centuries of scientific progress in understanding the structural and periodic patterns of the natural world, as well as our success in developing so many useful technologies that leverage these patterns, most humans are reasonably confident that our future will continue to unfold in a similar fashion.
The expectation that we will continue to find and utilize patterns, not only in the natural world but also in the realm of human and man-made system behavior, underlies the current enthusiasm for predictive analytics.
Amazon, Google, and Netflix have clearly demonstrated that predictions based on past user behavior can be profitable; fueling speculation that organizations of all types might benefit by uncovering the hidden patterns in their own data stores, and using these patterns to inform beneficial changes in their processes and systems.
Predictive applications can be used not only to find patterns that will increase sales and improve customer service, but also to improve the performance of complex systems. For example, even tiny changes to automated production processes, or catching anomalies before they turn into major malfunctions, can boost performance and profitability.
In short, it just makes sense to think that hidden patterns in the data that are being collected about our behavior, and about the behavior of our devices and systems, will be useful in predicting, influencing, and even changing, future behavior.
The concept is so compelling, and the data so voluminous, that a burgeoning array of new database and analytical software tools are coming to market, joining the existing statistical and decision modeling solutions, to advance the process of prediction. Some of these new tools are based on well-worn statistical paradigms.
Others feature algorithms developed in highly specialized realms of scientific research; while still others are being brought into commercial application, from research, into various types of machine learning. Some of the newest predictive analytic solutions offer bundles of algorithms and automated mechanisms (more algorithms), to evaluate their performance, and to identify the ones that best fit the available data and the desired predictions.
These algorithms owe their ability to work efficiently and rapidly to ongoing improvements in the software development frameworks that enable them, and in the ways that compute resources are scheduled and optimized.
The pace and intricacy of these developments make the field of predictive analytics one of the most complex and challenging subsets of the Big Data and advanced analytic market. There is also a very wide gap between the practitioners of predictive analytics—most of whom are statisticians, scientific researchers and data scientists—and the people who most want to use predictive analytics for commercial and industrial applications.
Bridging this gap with accurate information and clear communication is imperative if predictive applications are to satisfy business users’ expectations. The purpose of this report is to assist in building this bridge: first, by placing predictive analytics in a practical conceptual context for business people; then, by exploring several of the promising solutions that are coming to market.
This article was originally published prnewswire.com and can be viewed in full here


Archive
- October 2024(44)
- September 2024(94)
- August 2024(100)
- July 2024(99)
- June 2024(126)
- May 2024(155)
- April 2024(123)
- March 2024(112)
- February 2024(109)
- January 2024(95)
- December 2023(56)
- November 2023(86)
- October 2023(97)
- September 2023(89)
- August 2023(101)
- July 2023(104)
- June 2023(113)
- May 2023(103)
- April 2023(93)
- March 2023(129)
- February 2023(77)
- January 2023(91)
- December 2022(90)
- November 2022(125)
- October 2022(117)
- September 2022(137)
- August 2022(119)
- July 2022(99)
- June 2022(128)
- May 2022(112)
- April 2022(108)
- March 2022(121)
- February 2022(93)
- January 2022(110)
- December 2021(92)
- November 2021(107)
- October 2021(101)
- September 2021(81)
- August 2021(74)
- July 2021(78)
- June 2021(92)
- May 2021(67)
- April 2021(79)
- March 2021(79)
- February 2021(58)
- January 2021(55)
- December 2020(56)
- November 2020(59)
- October 2020(78)
- September 2020(72)
- August 2020(64)
- July 2020(71)
- June 2020(74)
- May 2020(50)
- April 2020(71)
- March 2020(71)
- February 2020(58)
- January 2020(62)
- December 2019(57)
- November 2019(64)
- October 2019(25)
- September 2019(24)
- August 2019(14)
- July 2019(23)
- June 2019(54)
- May 2019(82)
- April 2019(76)
- March 2019(71)
- February 2019(67)
- January 2019(75)
- December 2018(44)
- November 2018(47)
- October 2018(74)
- September 2018(54)
- August 2018(61)
- July 2018(72)
- June 2018(62)
- May 2018(62)
- April 2018(73)
- March 2018(76)
- February 2018(8)
- January 2018(7)
- December 2017(6)
- November 2017(8)
- October 2017(3)
- September 2017(4)
- August 2017(4)
- July 2017(2)
- June 2017(5)
- May 2017(6)
- April 2017(11)
- March 2017(8)
- February 2017(16)
- January 2017(10)
- December 2016(12)
- November 2016(20)
- October 2016(7)
- September 2016(102)
- August 2016(168)
- July 2016(141)
- June 2016(149)
- May 2016(117)
- April 2016(59)
- March 2016(85)
- February 2016(153)
- December 2015(150)