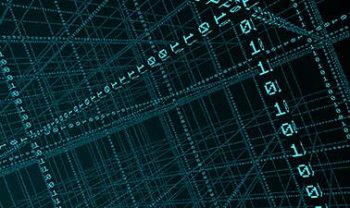
DataRobot, the pioneering architects of automated machine learning, today announced the general availability of DataRobot Time Series. Following an extensive collaboration with more than 75 customers and world-class data scientists, this latest breakthrough completely automates sophisticated time series modeling. Finally, frontline business people can quickly build highly accurate forecasts for a diverse array of time-dependent prediction opportunities.
Until now, handling time-dependency modeling required highly specialized expertise to even set up the problem correctly. Conventional modeling uses randomly selected records from a dataset to build and evaluate predictive models. For example, a representative sample of loan records and whether a customer defaulted. Each record is relatively similar.
Not so with time series. Temporal relationships matter. And there are so many potential ones to consider. Do you want to predict demand for a product three days from now? What day of the week is that? How does demand on that day of the week vary with demand on the other days? For the same day in previous weeks? Where does the week fall within the month? Does that matter? How about within the quarter? How is this month and this quarter comparing so far to last month and last quarter? The same month and quarter a year ago? Two years through 10 years ago? Is that a holiday in any location of interest and how does holiday performance differ? What promotions have you run and when did you run them? How have such promotions performed under similar temporal conditions?
Building on the 2017 acquisition of Nutonian, Inc. and its proven Eureqa modeling engine, DataRobot Time Series understands all these questions and how to set up the problem based on the answers. Then the DataRobot automation platform constructs and evaluates hundreds to thousands of different time series models and scores their performance – taking into account all the different temporal conditions to determine real world accuracy.
DataRobot Time Series beta customers, including Fortune 2000 retailers, banks, and hospital networks, have quickly built accurate models for staffing, inventory management, demand forecasting, financial applications, and more – all without the need for manual forecasting, specialized data science expertise, and custom coding.
“Forecasting underpins most critical business functions. If you can predict the future, you can usually win the game. But it is one of the hardest problems in data science. Since the Nutonian acquisition last May, we’ve been on a massive undertaking to combine Nutonian and DataRobot innovations into the best time series product in the world. This fourth version, which has been extensively tested by customers in production, automates a wide array of advanced best practices in areas like feature engineering and thereby achieves a whole new level of accuracy,” said Michael Schmidt, Chief Scientist, DataRobot.
This new version, which is available now, includes advanced machine learning models for forecasting, as well as essential time series methods like ARIMA and Facebook Prophet. Full API support helps AI engineers integrate modeling and prediction directly into business processes and applications.
“Time series machine learning has historically resisted automation,” says Srikant Datar, Professor of Business Administration and Faculty Chair of the Harvard Innovation Lab at Harvard Business School. “Having worked with DataRobot’s Time Series product for the past several months, including delivering real financial applications, I’m amazed at what is possible and how easily models can be built.”
Steward Health Care, the largest for-profit private hospital operator in the United States, is using DataRobot to significantly improve operational efficiency and reduce costs among their network of 38 hospitals across the nation. Sixty percent of hospital operational expenses come from staffing alone. With DataRobot’s improved forecasts for patient volume, Steward’s potential labor savings amount to $2 million by reducing hospital overstaffing by 1 percent for eight of the 38 hospitals in Steward’s network.
“We have data – a lot of data – and we want to use it to our advantage,” said Erin Sullivan, executive director of information systems and software development at Steward Health Care. “DataRobot has the tools to help us take historical data, manipulate it, and learn from it. We’ve already experienced tremendous cost and time savings with DataRobot, and these latest advancements will further transform how we forecast nurse staffing and patients’ length of stay—both of which will yield significant benefits for our hospital network.”


Archive
- October 2024(44)
- September 2024(94)
- August 2024(100)
- July 2024(99)
- June 2024(126)
- May 2024(155)
- April 2024(123)
- March 2024(112)
- February 2024(109)
- January 2024(95)
- December 2023(56)
- November 2023(86)
- October 2023(97)
- September 2023(89)
- August 2023(101)
- July 2023(104)
- June 2023(113)
- May 2023(103)
- April 2023(93)
- March 2023(129)
- February 2023(77)
- January 2023(91)
- December 2022(90)
- November 2022(125)
- October 2022(117)
- September 2022(137)
- August 2022(119)
- July 2022(99)
- June 2022(128)
- May 2022(112)
- April 2022(108)
- March 2022(121)
- February 2022(93)
- January 2022(110)
- December 2021(92)
- November 2021(107)
- October 2021(101)
- September 2021(81)
- August 2021(74)
- July 2021(78)
- June 2021(92)
- May 2021(67)
- April 2021(79)
- March 2021(79)
- February 2021(58)
- January 2021(55)
- December 2020(56)
- November 2020(59)
- October 2020(78)
- September 2020(72)
- August 2020(64)
- July 2020(71)
- June 2020(74)
- May 2020(50)
- April 2020(71)
- March 2020(71)
- February 2020(58)
- January 2020(62)
- December 2019(57)
- November 2019(64)
- October 2019(25)
- September 2019(24)
- August 2019(14)
- July 2019(23)
- June 2019(54)
- May 2019(82)
- April 2019(76)
- March 2019(71)
- February 2019(67)
- January 2019(75)
- December 2018(44)
- November 2018(47)
- October 2018(74)
- September 2018(54)
- August 2018(61)
- July 2018(72)
- June 2018(62)
- May 2018(62)
- April 2018(73)
- March 2018(76)
- February 2018(8)
- January 2018(7)
- December 2017(6)
- November 2017(8)
- October 2017(3)
- September 2017(4)
- August 2017(4)
- July 2017(2)
- June 2017(5)
- May 2017(6)
- April 2017(11)
- March 2017(8)
- February 2017(16)
- January 2017(10)
- December 2016(12)
- November 2016(20)
- October 2016(7)
- September 2016(102)
- August 2016(168)
- July 2016(141)
- June 2016(149)
- May 2016(117)
- April 2016(59)
- March 2016(85)
- February 2016(153)
- December 2015(150)