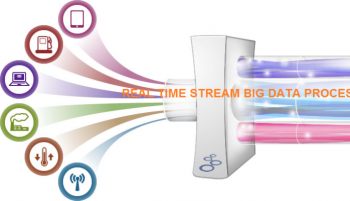
Companies today are looking for a data-driven competitive edge. That edge is increasingly derived from the timeliness of the data being processed and analysed.
For most companies, the methods and ways in which they are generating insights from data are developing at pace. Being able to capture and analyse data in real time is the new challenge.
Hadoop was the breakthrough that opened up a world of unstructured “Big Data” that enabled data scientists to start finding new patterns in data. This breakthrough allowed businesses to be transformed, accelerated research and development, and enabled accurate predictions in a way that we had not been able to do before.
The first wave of big data projects focused on analysing historical data sets. While the volume and variety of data being analysed were on a massive scale, it was always data that was recorded after the event and ingested into a data store like HDFS. Times have moved on, and analysis of historical data alone is no longer likely to deliver the competitive advantage that business strives to find.
The approach to analytics is still valid, but increasingly the answers that companies are looking for are held in data at the moment it is created.
Companies need to be able to access, process and analyse data in real-time. The industrial IoT and the Internet of Anything (IoAT) are providing new sources of data streams that provide telemetry or information on devices that monitor systems, people, vehicles, machines, and equipment in real-time.
As an example, grabbing data from thousands of sensors in real-time enables businesses to make by-the-second decisions to improve efficiencies, deliver goods faster and even avoid accidents or failures.
Real-time analytics enables big data style analysis and insight to be applied to processes, procedures and production as they occur. However, a word of caution is required. Just like the early days of big data, if not done correctly, a lot of time, resource and money can be wasted. With these pitfalls in mind, here are five important points you need to consider when working with data in real-time.
1) Use the Right Technology. Get help from experts to do so. Analysing thousands of real-time data streams is not a simple task, and neither can it be achieved with a one-size-fits-all approach. Reading data science message boards, you will see data scientists ask questions like which is better, Apache Kafka or NiFi or Storm. The truth is that these different components of the Apache project are actually complementary and dependent on your use case. You may even need to combine them all. An experienced big data provider like Cloudera can help companies integrate the technologies that best suit their own data sources.
2) Customer Touchpoints. In addition to connected devices, most companies are using increasing numbers of SaaS applications to service their own staff and customer interactions. Effectively, this means that customers are interacting with businesses across multiple disparate touch points. Much of this data can be valuable to real-time analytics especially if it can be applied to supply chain decisions on the fly. These touch points need to be identified and integrated into a real-time data teaming framework.
3) Preserve & Understand Object Relationships. If data objects in different systems have relationship dependencies, this is something that can be maintained in traditional ETL in a data store. Preserving these dependencies and relationship between objects streaming in real-time from two different data sources is much harder to do. This ties back to point one above and ensuring you use the right technology for the data sources you stream.
4) Data and Storage Management. Building and running infrastructure for a big data installation has always come with unique challenges. Adding massive streams of data in real-time increases this challenge further. You need a data management strategy and culture that maximises the efficiency of the data you stream. As an example, Twitter developed analytics management tools to identify fake and fraudulent accounts and remove them from its real-time data analytics streams.
In addition, you also need storage that can cope with unexpected volumes being generated from real-time data sources. The cloud (be it public, private or hybrid) becomes a great option here as it can provide you with the flexibility, scalability and elasticity you need in order to cope with huge volumes of varying data cost-effectively.
5) Data Integrity. Real-time streaming comes with new data integrity challenges. When you ingest data into something like HDFS, part of the ingest process can include data cleansing. This “luxury” is not possible with real-time data analytics. The quality of the data streams needs to be high at the source. This is not just a technical issue. Promoting a culture of data accountability across staff at all levels can help with the integrity of data at the source but requires that top management subscribes to the benefits they will see from successful real-time analytics.
Your business will benefit from real-time data analytics “done right” but “done wrong”, can have an even stronger negative effect. Working with the right people, companies and technologies will accelerate your time to success in the world of real-time analytics.
For more information, please click here.


Archive
- October 2024(44)
- September 2024(94)
- August 2024(100)
- July 2024(99)
- June 2024(126)
- May 2024(155)
- April 2024(123)
- March 2024(112)
- February 2024(109)
- January 2024(95)
- December 2023(56)
- November 2023(86)
- October 2023(97)
- September 2023(89)
- August 2023(101)
- July 2023(104)
- June 2023(113)
- May 2023(103)
- April 2023(93)
- March 2023(129)
- February 2023(77)
- January 2023(91)
- December 2022(90)
- November 2022(125)
- October 2022(117)
- September 2022(137)
- August 2022(119)
- July 2022(99)
- June 2022(128)
- May 2022(112)
- April 2022(108)
- March 2022(121)
- February 2022(93)
- January 2022(110)
- December 2021(92)
- November 2021(107)
- October 2021(101)
- September 2021(81)
- August 2021(74)
- July 2021(78)
- June 2021(92)
- May 2021(67)
- April 2021(79)
- March 2021(79)
- February 2021(58)
- January 2021(55)
- December 2020(56)
- November 2020(59)
- October 2020(78)
- September 2020(72)
- August 2020(64)
- July 2020(71)
- June 2020(74)
- May 2020(50)
- April 2020(71)
- March 2020(71)
- February 2020(58)
- January 2020(62)
- December 2019(57)
- November 2019(64)
- October 2019(25)
- September 2019(24)
- August 2019(14)
- July 2019(23)
- June 2019(54)
- May 2019(82)
- April 2019(76)
- March 2019(71)
- February 2019(67)
- January 2019(75)
- December 2018(44)
- November 2018(47)
- October 2018(74)
- September 2018(54)
- August 2018(61)
- July 2018(72)
- June 2018(62)
- May 2018(62)
- April 2018(73)
- March 2018(76)
- February 2018(8)
- January 2018(7)
- December 2017(6)
- November 2017(8)
- October 2017(3)
- September 2017(4)
- August 2017(4)
- July 2017(2)
- June 2017(5)
- May 2017(6)
- April 2017(11)
- March 2017(8)
- February 2017(16)
- January 2017(10)
- December 2016(12)
- November 2016(20)
- October 2016(7)
- September 2016(102)
- August 2016(168)
- July 2016(141)
- June 2016(149)
- May 2016(117)
- April 2016(59)
- March 2016(85)
- February 2016(153)
- December 2015(150)