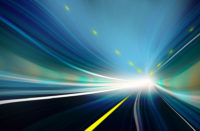
The abundance of big new data types is creating a feeding frenzy among companies as they gorge themselves at a veritable smorgasbord of information. In every industry, entrepreneurs are trying out new ways to harness big data for competitive advantage. But going from data science project to actionable insight is not as easy as it sounds, and the smart approach demands pragmatic solutions.
There are good reasons to be excited about the big data phenomenon. We’re smack dab in the middle of the hockey-stick curve when it comes to data generation, and the curve will only steepen if the promised glut of 1s and 0s from the IoT materializes. And while only a small fraction of the data we generate today gets analyzed (less than 1 percent by some estimates), lots of smart people wake up every morning determined to figure out how to make sense of it all.
To be sure, we are at the cross-roads of the digital revolution. We may have thought we were living in a digital world, what with the popularization of things like smart phones, XML, DVDs, and MP3s over the past 20 years. But there still exist huge swaths of our everyday lives that have yet to be “optimized,” or converted from analog to digital formats, as it were (you can an LP from a record aficionado’s cold, dead hands). The rush is on to be the next Uber and disrupt whole industries–and there will be more disruptions.
But in this rush to leverage big data to topple old forms of commerce and be the new king of industry, there will be many mistakes made. While the Silicon Valley seems to possess an infinite supply of courage to reimagine the Next Big Thing, there are, frankly, many wrong ways to approach big data.
This is especially true for existing businesses that are trying to do data science for the first time. While executive strategists constantly implore companies to “think like startups,” the fact is the risks of disrupting a successful line of business with unproven approaches are far too great. Instead, the new data-driven process must be proven to work and carefully merged into production. Anything else is folly.
Scoping Out Analytics’ Role
Bill Schmarzo, author of the recently published book “Big Data MBA,” encourages would-be big data practitioners to slow down and think through the basics. “First off, we explain to clients what data science is,” the EMC executive, who’s been called “The Dean of Big Data,” told Datanami recently. “Data science is identifying those variable and metrics that might be better predictors of performance.”
The field of data science might have the reputation of being a black art that only a few can succeed at, but Schmarzo takes a much broader view, and asks business executives to take an active role in finding variables that might be better predictors of performance. Once those insights are identified, it’s up to the data scientists to figure out whether they’re valuable.Schmarzo_SAM
“No matter what industry we’re in, the data scientists can do data science. They can bring the skill sets they have and the business people can collaborate to help us brainstorm those variables and metrics,” he says.
All too often, people get caught up in the latest technology and buzzwords in the big data space. But if people start from the business side of the equation, and then work back toward the technology, that keep them from going astray into the big data jungle.
“Do you need to know Hadoop as a business person? Heck no!” Schmarzo says. “Don’t worry if you can spell it right or wrong. You just need to know that data now is an asset, that you can go out and get whatever you want – whether structured or unstructured, whether it’s off sensors or PDF files or screen scraping off a website.
“The technology enables us to think differently and that’s why it’s cultural challenge,” he continues. “We need to help the business think differently about how I can get value out of data. The thing that’s really hard for organizations to get their heads around is the big data technologies are 20 to 50x cheaper than traditional data warehousing technology…. But until I know what decision I’m trying to make, until I know what data sources I’m trying to mine, until I know what kind of algorithms I want to run–that tells me what technology I need.”
Action-Packed Data
Big data is often described using the three Vs: volume, variety, and velocity. But with huge object oriented file systems that can keep yottabytes in a single addressable space, and real-time message brokers like Apache Kafka that can scale to infinity (and possibly beyond!) most data analytic professionals today say the real challenge is the variety. And that means unstructured data.
While images, video, and text are among the fastest growing data types, using big data algorithms to turn unstructured ore into structured gold is easier said than done. And without a clearly defined data strategy, companies risk spending big money on text or image processing setups, only to find themselves searching for how to turn that into business innovation.
“If you can’t integrate the insight with the business process, you’re not going to get any value from the insight,” says Scott Crowder, the CTO of IBM Systems and VP of technical strategy and transformation for IBM (NYSE: IBM).
IBM supplies IT products and services to some of the world’s largest organizations in the healthcare, financial services, and oil and gas industries. They already spend millions with IBM, and are keen to use data analytics to drive their businesses forward. But just having the insight from voice or image or text analytics without a way to actually use the insight gleaned from the data is essentially worthless, he says.
“You’ve got to ingest the data, you’ve got to gain insights from the data, but you also need to then decide what action you’re going to take from that insight, and actually take the action,” Crowder says. “You can have the best insight in the world but if you’re infrastructure is keeping you from taking real time action, you’re wasting a lot of money.”
Up Next: Neural Networking
IBM is bullish on the prospects of its Power Systems and OpenPower ecosystems to help customers push their data science and cognitive computing strategies from the drawing board into actual production.neural networks_4
While the Power hardware costs more than Intel (NYSE: INTC) X86 hardware upfront, Crowder says Power’s 4x performance advantages in memory bandwidth, cache, and threads—not to mention its coherent acceleration processor interface (CAPI) and integration with field programmable gate arrays (FPGAs) and graphic processing units (GPUs)–give it the edge in the evolving cognitive landscapes.
“Image analytics running on commodity hardware is just too slow,” Crowder says. “Almost all deep learning at scale—whether its image or voice or text analytics–is leveraging acceleration of some sort, whether GPU or FPGA.”
Crowder is keeping his eye on a new group of Silicon Valley startups that are building the next generation of chips designed specifically to handle emerging big data workloads, specifically deep learning. “There’s another generation of innovation that’s coming that’s basically neural net acceleration in hardware,” he says. “These days you can’t shake a stick without hitting one of these hardware neural network startups.”
This article was originally published on www.datanami.com and can be viewed in full


Archive
- October 2024(44)
- September 2024(94)
- August 2024(100)
- July 2024(99)
- June 2024(126)
- May 2024(155)
- April 2024(123)
- March 2024(112)
- February 2024(109)
- January 2024(95)
- December 2023(56)
- November 2023(86)
- October 2023(97)
- September 2023(89)
- August 2023(101)
- July 2023(104)
- June 2023(113)
- May 2023(103)
- April 2023(93)
- March 2023(129)
- February 2023(77)
- January 2023(91)
- December 2022(90)
- November 2022(125)
- October 2022(117)
- September 2022(137)
- August 2022(119)
- July 2022(99)
- June 2022(128)
- May 2022(112)
- April 2022(108)
- March 2022(121)
- February 2022(93)
- January 2022(110)
- December 2021(92)
- November 2021(107)
- October 2021(101)
- September 2021(81)
- August 2021(74)
- July 2021(78)
- June 2021(92)
- May 2021(67)
- April 2021(79)
- March 2021(79)
- February 2021(58)
- January 2021(55)
- December 2020(56)
- November 2020(59)
- October 2020(78)
- September 2020(72)
- August 2020(64)
- July 2020(71)
- June 2020(74)
- May 2020(50)
- April 2020(71)
- March 2020(71)
- February 2020(58)
- January 2020(62)
- December 2019(57)
- November 2019(64)
- October 2019(25)
- September 2019(24)
- August 2019(14)
- July 2019(23)
- June 2019(54)
- May 2019(82)
- April 2019(76)
- March 2019(71)
- February 2019(67)
- January 2019(75)
- December 2018(44)
- November 2018(47)
- October 2018(74)
- September 2018(54)
- August 2018(61)
- July 2018(72)
- June 2018(62)
- May 2018(62)
- April 2018(73)
- March 2018(76)
- February 2018(8)
- January 2018(7)
- December 2017(6)
- November 2017(8)
- October 2017(3)
- September 2017(4)
- August 2017(4)
- July 2017(2)
- June 2017(5)
- May 2017(6)
- April 2017(11)
- March 2017(8)
- February 2017(16)
- January 2017(10)
- December 2016(12)
- November 2016(20)
- October 2016(7)
- September 2016(102)
- August 2016(168)
- July 2016(141)
- June 2016(149)
- May 2016(117)
- April 2016(59)
- March 2016(85)
- February 2016(153)
- December 2015(150)