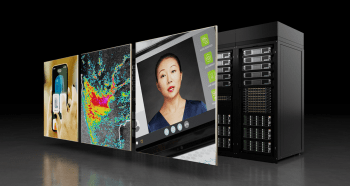
Two months after their debut sweeping MLPerf inference benchmarks, NVIDIA H100 Tensor Core GPUs set world records across enterprise Artificial Intelligence (AI) workloads in the industry group’s latest tests of AI training.
Together, the results show H100 is the best choice for users who demand utmost performance when creating and deploying advanced AI models.
MLPerf is the industry standard for measuring AI performance. It is backed by a broad group that includes Amazon, Arm, Baidu, Google, Harvard University, Intel, Meta, Microsoft, Stanford University and University of Toronto.
In a related MLPerf benchmark also released recently, NVIDIA A100 Tensor Core GPUs raised the bar they set last year in high-performance computing (HPC).
H100 GPUs (aka Hopper) raised the bar in per-accelerator performance in MLPerf Training. They delivered up to 6.7x more performance than previous-generation GPUs when they were first submitted on MLPerf training. By the same comparison, today’s A100 GPUs pack 2.5x more muscle, thanks to advances in software.
Due in part to its Transformer Engine, Hopper excelled in training the popular BERT model for natural language processing. It is among the largest and most performance-hungry of the MLPerf AI models.
MLPerf gives users the confidence to make informed buying decisions because the benchmarks cover today’s most popular AI workloads—computer vision, natural language processing, recommendation systems, reinforcement learning and more. The tests are peer reviewed, so users can rely on their results.
A100 GPUs Hit New Peak in HPC
In the separate suite of MLPerf HPC benchmarks, A100 GPUs swept all tests of training AI models in demanding scientific workloads run on supercomputers. The results show the NVIDIA AI platform’s ability to scale to the world’s toughest technical challenges.
For example, A100 GPUs trained AI models in the CosmoFlow test for astrophysics 9x faster than the best results two years ago in the first round of MLPerf HPC. In that same workload, the A100 also delivered up to a whopping 66x more throughput per chip than an alternative offering.
The HPC benchmarks train models for work in astrophysics, weather forecasting and molecular dynamics. They are among many technical fields, like drug discovery, adopting AI to advance science.
Supercomputer centres in Asia, Europe and the U.S. participated in the latest round of the MLPerf HPC tests. In its debut on the DeepCAM benchmarks, Dell Technologies showed strong results using NVIDIA A100 GPUs.
An Unparalleled Ecosystem
In the enterprise AI training benchmarks, a total of 11 companies, including the Microsoft Azure cloud service, made submissions using NVIDIA A100, A30 and A40 GPUs. System makers including ASUS, Dell Technologies, Fujitsu, GIGABYTE, Hewlett Packard Enterprise, Lenovo and Supermicro used a total of nine NVIDIA-Certified Systems for their submissions.
In the latest round, at least three companies joined NVIDIA in submitting results on all eight MLPerf training workloads. That versatility is important because real-world applications often require a suite of diverse AI models.
NVIDIA partners participate in MLPerf because they know it is a valuable tool for customers evaluating AI platforms and vendors.
Under the Hood
The NVIDIA AI platform provides a full stack from chips to systems, software and services. That enables continuous performance improvements over time.
For example, submissions in the latest HPC tests applied a suite of software optimisations and techniques described in a technical article. Together they slashed runtime on one benchmark by 5x, to just 22 minutes from 101 minutes.
A second article describes how NVIDIA optimised its platform for enterprise AI benchmarks. For example, we used NVIDIA DALI to efficiently load and pre-process data for a computer vision benchmark.
All the software used in the tests is available from the MLPerf repository, so anyone can get these world-class results. NVIDIA continuously folds these optimizations into containers available on NGC, a software hub for GPU applications.


Archive
- October 2024(44)
- September 2024(94)
- August 2024(100)
- July 2024(99)
- June 2024(126)
- May 2024(155)
- April 2024(123)
- March 2024(112)
- February 2024(109)
- January 2024(95)
- December 2023(56)
- November 2023(86)
- October 2023(97)
- September 2023(89)
- August 2023(101)
- July 2023(104)
- June 2023(113)
- May 2023(103)
- April 2023(93)
- March 2023(129)
- February 2023(77)
- January 2023(91)
- December 2022(90)
- November 2022(125)
- October 2022(117)
- September 2022(137)
- August 2022(119)
- July 2022(99)
- June 2022(128)
- May 2022(112)
- April 2022(108)
- March 2022(121)
- February 2022(93)
- January 2022(110)
- December 2021(92)
- November 2021(107)
- October 2021(101)
- September 2021(81)
- August 2021(74)
- July 2021(78)
- June 2021(92)
- May 2021(67)
- April 2021(79)
- March 2021(79)
- February 2021(58)
- January 2021(55)
- December 2020(56)
- November 2020(59)
- October 2020(78)
- September 2020(72)
- August 2020(64)
- July 2020(71)
- June 2020(74)
- May 2020(50)
- April 2020(71)
- March 2020(71)
- February 2020(58)
- January 2020(62)
- December 2019(57)
- November 2019(64)
- October 2019(25)
- September 2019(24)
- August 2019(14)
- July 2019(23)
- June 2019(54)
- May 2019(82)
- April 2019(76)
- March 2019(71)
- February 2019(67)
- January 2019(75)
- December 2018(44)
- November 2018(47)
- October 2018(74)
- September 2018(54)
- August 2018(61)
- July 2018(72)
- June 2018(62)
- May 2018(62)
- April 2018(73)
- March 2018(76)
- February 2018(8)
- January 2018(7)
- December 2017(6)
- November 2017(8)
- October 2017(3)
- September 2017(4)
- August 2017(4)
- July 2017(2)
- June 2017(5)
- May 2017(6)
- April 2017(11)
- March 2017(8)
- February 2017(16)
- January 2017(10)
- December 2016(12)
- November 2016(20)
- October 2016(7)
- September 2016(102)
- August 2016(168)
- July 2016(141)
- June 2016(149)
- May 2016(117)
- April 2016(59)
- March 2016(85)
- February 2016(153)
- December 2015(150)