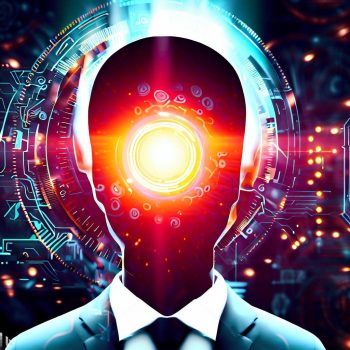
Written By: Remus Lim, Vice President, Asia Pacific & Japan, at Cloudera
The age of artificial intelligence (AI) is here. Generative AI models, like ChatGPT and DALL-E 2, have skyrocketed in popularity, partly due to their ease of use and public availability. AI Appreciation Day, held every 16 July, serves as a reminder of how fast AI has broken into the mainstream consciousness in the last few years.
With generative AI being able to assist with answering queries, organising data and executing repetitive tasks, enterprises too are increasingly turning towards AI to supercharge productivity and drive innovation.
From accelerating creative work, to optimising supply chains, and designing medical drugs—AI is driving society towards a new industrial revolution. IDC research recently revealed that two out of three organizations in Asia Pacific are exploring or have invested in generative AI technologies in 2023.
Inaccuracies and Bias Are Pressing Issues
As AI enters the mainstream, questions are rising on the trust we can place in these tools and if there are enough safeguards. While AI-generated deepfakes are being used widely to perpetrate disinformation, intellectual property and personal information continue to be compromised on platforms like ChatGPT.
More concerning are AI hallucinations. Users of AI today often encounter instances where generative models produce inaccurate or irrelevant results that appear confident and well-phrased enough to be easily believable.
Data bias is another worrying issue that threatens to magnify AI’s negative impact on society. Humans possess many forms of ingrained biases, like confirmation bias, that are magnified in large data sets. When this biased data is used to train AI models, their outputs will also present bias.
For example, image generation models which are not well trained can present outputs with outdated stereotypes. Examples are images of an older man in a suit when asked to generate a CEO, or a young woman when asked to visualise a flight attendant.
These concerns about AI can have severe implications on enterprises. For example, a medical imaging AI designed to diagnose patients could be useful at diagnosing patients with common conditions, but due to training data biased against unrepresented groups, end up prescribing ineffectual or harmful treatments to patients with rare health conditions.
If left unchecked now, AI could perpetrate unfairness across society—in hiring, loan disbursements, school admissions. and more. This can then lead to loss of trust, financial losses. or worse.
Addressing Inaccuracy and Bias as AI Technologies Continue to Sprawl
Addressing today’s emerging AI issues requires us to take a closer look at its building blocks—data. Most issues seen in generative AI are caused by training them on ‘untrusted’ data that is erroneous, outdated, or not diverse enough for its use case.
“Users of AI today often encounter instances where generative models produce inaccurate or irrelevant results that appear confident and well-phrased enough to be easily believable.”
As this ‘untrusted’ data is passed through processes and algorithms to produce results, this may distort the data even more, magnifying inaccuracies and bias in AI’s outputs. Many enterprises also lack visibility on the traceability of their training data, which prevents users from understanding how it changes over time and how AI derives its results.
For AI applications, including generative AI, to be successful, they need to be trusted. Enterprises must have confidence in their data and its quality. This starts with implementing good data governance practices, which ensures that data is clean, securely managed, traceable, and ready for movement and analysis across environments.
Tackling bias requires organisations to re-look at their data strategies and how they plan the scope of their data. Training datasets for AI must contain sample sizes representative enough for its planned use case. Assigning humans to continuously audit training data and decisions made by AI for bias is essential to making iterative improvements. Organisations must also ensure the diversity of human teams, which will help address issues like confirmation bias.
Enhancing Accuracy
This careful selection and management of datasets also helps enterprises enhance the accuracy of results as they use AI. Enterprises seeking to create their versions of ChatGPT and large language models must use contextually-relevant data, rather than models trained on publicly available data, to ensure the best relevant results to their use case. For example, for AI to help enterprises improve customer service, its training data must be relevant or derived from the exact areas of focus.
To better handle the vast amounts of data used by AI, organisations can use data management platforms to facilitate the cleaning, governance, tracking, auditing, and movement of data—wherever it resides. Platforms with security, observability, and traceability built-in as features also help enterprises manage their proprietary data sources securely and make AI models more explainable.
Creating Safer and Better AI Requires Open Collaboration
To fight bias, inaccuracy, and misuse in AI at scale, AI players, business sectors, and governments need to work together. Setting up more comprehensive regulations governing AI, common technology and ethical standards, and open, collaborative workflows is key to success.
For instance, open standards can give financial services organisations the means to detect AI-generated content being used for fraudulent, synthetic applications for bank accounts. In turn, finance players can share information they gather on fraud, abuse, bias and other trends driven by AI to the wider public—advising better development of safeguards and regulations.
Recently, 60 industry leaders, including Google, Microsoft, and Meta, formed an alliance with the Singapore authorities to tackle pressing issues in AI such as bias and misuse—and to create a neutral platform for collaboration on governing AI.
“Tackling bias requires organisations to re-look at their data strategies and how they plan the scope of their data. Training datasets for AI must contain sample sizes representative enough for its planned use case. Assigning humans to continuously audit training data and decisions made by AI for bias is essential to making iterative improvements.”
Alliances like these are a great start to tackling AI issues, and we hope to see such efforts replicated across regions. However, it takes more than agreements and discussion papers to enact meaningful change in the long run.
Translating plans into action requires organizations to adopt modern data architectures that facilitate open data sharing across environments, better data governance, and faster speed to insight. These data technologies are crucial to forming a foundation for safer and better AI development, and key to building trust in new game-changing AI applications.


Archive
- October 2024(44)
- September 2024(94)
- August 2024(100)
- July 2024(99)
- June 2024(126)
- May 2024(155)
- April 2024(123)
- March 2024(112)
- February 2024(109)
- January 2024(95)
- December 2023(56)
- November 2023(86)
- October 2023(97)
- September 2023(89)
- August 2023(101)
- July 2023(104)
- June 2023(113)
- May 2023(103)
- April 2023(93)
- March 2023(129)
- February 2023(77)
- January 2023(91)
- December 2022(90)
- November 2022(125)
- October 2022(117)
- September 2022(137)
- August 2022(119)
- July 2022(99)
- June 2022(128)
- May 2022(112)
- April 2022(108)
- March 2022(121)
- February 2022(93)
- January 2022(110)
- December 2021(92)
- November 2021(107)
- October 2021(101)
- September 2021(81)
- August 2021(74)
- July 2021(78)
- June 2021(92)
- May 2021(67)
- April 2021(79)
- March 2021(79)
- February 2021(58)
- January 2021(55)
- December 2020(56)
- November 2020(59)
- October 2020(78)
- September 2020(72)
- August 2020(64)
- July 2020(71)
- June 2020(74)
- May 2020(50)
- April 2020(71)
- March 2020(71)
- February 2020(58)
- January 2020(62)
- December 2019(57)
- November 2019(64)
- October 2019(25)
- September 2019(24)
- August 2019(14)
- July 2019(23)
- June 2019(54)
- May 2019(82)
- April 2019(76)
- March 2019(71)
- February 2019(67)
- January 2019(75)
- December 2018(44)
- November 2018(47)
- October 2018(74)
- September 2018(54)
- August 2018(61)
- July 2018(72)
- June 2018(62)
- May 2018(62)
- April 2018(73)
- March 2018(76)
- February 2018(8)
- January 2018(7)
- December 2017(6)
- November 2017(8)
- October 2017(3)
- September 2017(4)
- August 2017(4)
- July 2017(2)
- June 2017(5)
- May 2017(6)
- April 2017(11)
- March 2017(8)
- February 2017(16)
- January 2017(10)
- December 2016(12)
- November 2016(20)
- October 2016(7)
- September 2016(102)
- August 2016(168)
- July 2016(141)
- June 2016(149)
- May 2016(117)
- April 2016(59)
- March 2016(85)
- February 2016(153)
- December 2015(150)