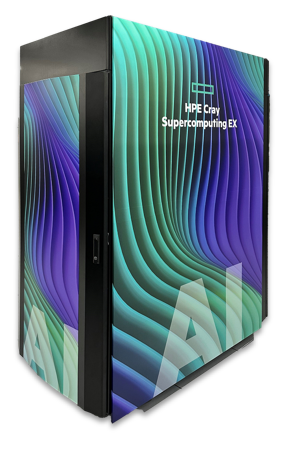
Hewlett Packard Enterprise (HPE) announced at GTC 2024 updates to one of the industry’s most comprehensive Artificial Intelligence (AI)-native portfolios to advance the operationalisation of generative AI (GenAI), deep learning, and machine learning (ML) applications.
These updates include:
- Availability of two HPE and NVIDIA co-engineered full-stack GenAI solutions.
- A preview of HPE Machine Learning Inference Software.
- An enterprise retrieval-augmented generation (RAG) reference architecture.
- Support to develop future products based on the new NVIDIA Blackwell platform.
“To deliver on the promise of GenAI and effectively address the full AI lifecycle, solutions must be hybrid by design,” said Antonio Neri, President and CEO at HPE. “From training and tuning models on-premises, in a colocation facility or the public cloud, to inferencing at the edge, AI is a hybrid cloud workload. HPE and NVIDIA have a long history of collaborative innovation, and we will continue to deliver co-designed AI software and hardware solutions that help our customers accelerate the development and deployment of GenAI from concept into production.”
“Generative AI can turn data from connected devices, data centres, and clouds into insights that can drive breakthroughs across industries,” said Jensen Huang, Founder of and CEO at NVIDIA. “Our growing collaboration with HPE will enable enterprises to deliver unprecedented productivity by leveraging their data to develop and deploy new AI applications to transform their businesses.”
Supercomputing-Powered GenAI Training and Tuning
Announced at SC23, HPE’s supercomputing solution for generative AI is now available to order for organizations seeking a preconfigured and pretested full-stack solution for the development and training of large AI models. Purpose-built to help customers accelerate GenAI and deep learning projects, the turnkey solution is powered by NVIDIA and can support up to 168 NVIDIA GH200 Grace Hopper Superchips.
The solution enables large enterprises, research institutions, and government entities to streamline the model development process with an AI/ML software stack that helps customers accelerate GenAI and deep learning projects, including LLMs, recommender systems, and vector databases. Delivered with services for installation and set-up, this turnkey solution is designed for use in AI research centers and large enterprises to realize improved time-to-value and speed up training by 2-3X. (For more information or to order it today, visit HPE’s supercomputing solution for generative AI.)
Enterprise-class GenAI Tuning and Inference
Previewed at Discover Barcelona 2023, HPE’s enterprise computing solution for generative AI is now available to customers directly or through HPE GreenLake with a flexible and scalable pay-per-use model.
Co-engineered with NVIDIA, the pre-configured fine-tuning and inference solution is designed to reduce ramp-up time and costs by offering the right compute, storage, software, networking, and consulting services that organisations need to produce GenAI applications. The AI-native full-stack solution gives businesses the speed, scale and control necessary to tailor foundational models using private data and deploy GenAI applications within a hybrid cloud model.
Featuring a high-performance AI compute cluster and software from HPE and NVIDIA, the solution is ideal for lightweight fine-tuning of models, RAG, and scale-out inference. The fine-tuning time for a 70 billion parameter Llama 2 model running this solution decreases linearly with node count, taking six minutes on a 16-node system. The speed and performance enable customers to realise faster time-tovalue by improving business productivity with AI applications like virtual assistants, intelligent chatbots, and enterprise search.
Powered by HPE ProLiant DL380a Gen11 servers, the solution is pre-configured with NVIDIA GPUs, the NVIDIA Spectrum-X Ethernet networking platform, and NVIDIA BlueField-3 DPUs. The solution is enhanced by HPE’s machine learning platform and analytics software, NVIDIA AI Enterprise 5.0 software with new NVIDIA NIM microservice for optimised inference of generative AI models, as well as NVIDIA NeMo Retriever and other data science and AI libraries.
To address the AI skills gap, HPE Services experts will help enterprises design, deploy, and manage the solution, which includes applying appropriate model tuning techniques.
HPE and NVIDIA: From Prototype to Productivity
HPE and NVIDIA are collaborating on software solutions that will help enterprises take the next step by turning AI and ML proofs-of-concept into production applications. Available to HPE customers as a technology preview, HPE Machine Learning Inference Software will allow enterprises to rapidly and securely deploy ML models at scale. The new offering will integrate with NVIDIA NIM to deliver NVIDIA-optimised foundation models using pre-built containers.
To assist enterprises that need to rapidly build and deploy GenAI applications that feature private data, HPE developed a reference architecture for enterprise RAG, available today, that is based on the NVIDIA NeMo Retriever microservice architecture. The offering consists of a comprehensive data foundation from HPE Ezmeral Data Fabric Software and HPE GreenLake for File Storage. The new reference architecture will offer businesses a blueprint to create customized chatbots, generators, or copilots.
To aid in data preparation, AI training, and inferencing, the solution merges the full spectrum of opensource tools and solutions from HPE Ezmeral Unified Analytics Software and HPE’s AI software, which includes HPE Machine Learning Data Management Software, HPE Machine Learning Development Environment Software, and the new HPE Machine Learning Inference Software. HPE’s AI software is available on both HPE’s supercomputing and enterprise computing solutions for generative AI to provide a consistent environment for customers to manage their GenAI workloads.
Next-Gen Solutions Built on NVIDIA Blackwell Platform
HPE will develop future products based on the newly announced NVIDIA Blackwell platform, which incorporates a second-generation Transformer Engine to accelerate GenAI workloads. Additional details and availability for forthcoming HPE products featuring the NVIDIA GB200 Grace Blackwell Superchip, the HGX B200, and the HGXB100 will be announced in the future


Archive
- October 2024(44)
- September 2024(94)
- August 2024(100)
- July 2024(99)
- June 2024(126)
- May 2024(155)
- April 2024(123)
- March 2024(112)
- February 2024(109)
- January 2024(95)
- December 2023(56)
- November 2023(86)
- October 2023(97)
- September 2023(89)
- August 2023(101)
- July 2023(104)
- June 2023(113)
- May 2023(103)
- April 2023(93)
- March 2023(129)
- February 2023(77)
- January 2023(91)
- December 2022(90)
- November 2022(125)
- October 2022(117)
- September 2022(137)
- August 2022(119)
- July 2022(99)
- June 2022(128)
- May 2022(112)
- April 2022(108)
- March 2022(121)
- February 2022(93)
- January 2022(110)
- December 2021(92)
- November 2021(107)
- October 2021(101)
- September 2021(81)
- August 2021(74)
- July 2021(78)
- June 2021(92)
- May 2021(67)
- April 2021(79)
- March 2021(79)
- February 2021(58)
- January 2021(55)
- December 2020(56)
- November 2020(59)
- October 2020(78)
- September 2020(72)
- August 2020(64)
- July 2020(71)
- June 2020(74)
- May 2020(50)
- April 2020(71)
- March 2020(71)
- February 2020(58)
- January 2020(62)
- December 2019(57)
- November 2019(64)
- October 2019(25)
- September 2019(24)
- August 2019(14)
- July 2019(23)
- June 2019(54)
- May 2019(82)
- April 2019(76)
- March 2019(71)
- February 2019(67)
- January 2019(75)
- December 2018(44)
- November 2018(47)
- October 2018(74)
- September 2018(54)
- August 2018(61)
- July 2018(72)
- June 2018(62)
- May 2018(62)
- April 2018(73)
- March 2018(76)
- February 2018(8)
- January 2018(7)
- December 2017(6)
- November 2017(8)
- October 2017(3)
- September 2017(4)
- August 2017(4)
- July 2017(2)
- June 2017(5)
- May 2017(6)
- April 2017(11)
- March 2017(8)
- February 2017(16)
- January 2017(10)
- December 2016(12)
- November 2016(20)
- October 2016(7)
- September 2016(102)
- August 2016(168)
- July 2016(141)
- June 2016(149)
- May 2016(117)
- April 2016(59)
- March 2016(85)
- February 2016(153)
- December 2015(150)