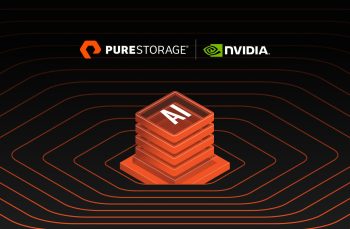
Pure Storage®, the IT pioneer that delivers the world’s most advanced data storage technology and services, has announced new validated reference architectures for running generative AI use cases, including a new NVIDIA OVX-ready validated reference architecture.
As a leader in AI, Pure Storage, in collaboration with NVIDIA, is arming global customers with a proven framework to manage the high-performance data and compute requirements they need to drive successful AI deployments.
“Pure Storage recognised the rising demand for AI early on, delivering an efficient, reliable, and high-performance platform for the most advanced AI deployments. Embracing our long-standing collaboration with NVIDIA, the latest validated AI reference architectures and generative AI proofs of concept emerge as pivotal components for global enterprises in unraveling the complexities of the AI puzzle,” said Rob Lee, Chief Technology Officer at Pure Storage.
“NVIDIA’s AI platform reference architectures are enhanced by Pure’s simple, efficient, and reliable data infrastructure, delivering comprehensive solutions for enterprises navigating the complexities of AI, data analytics, and advanced computing. Pure’s latest validated reference architectures and proofs of concept are helping drive AI innovation, providing businesses with the tools needed to unlock new possibilities and drive transformative outcomes,” added Bob Pette, Vice President of Enterprise Platforms at NVIDIA.
Pure Storage Is Delivering the Latest Tech in AI
Building on this collaboration with NVIDIA, Pure Storage delivers the latest technologies to meet the rapidly growing demand for AI across today’s enterprises. New validated designs and proofs of concept include:
- Retrieval-Augmented Generation (RAG) Pipeline for AI Inference To improve the accuracy, currency, and relevance of Inference capabilities for large language models (LLMs), Pure Storage created a RAG pipeline leveraging NVIDIA NeMo Retriever microservices and NVIDIA GPUs and Pure Storage for all-flash enterprise storage. As a result, Pure Storage accelerates time to insight for enterprises using their internal data for AI training, ensuring the use of their latest data and eliminating the need for constant retraining of LLMs.
- Certified NVIDIA OVX Server Storage Reference Architecture. Pure Storage has achieved OVX Server Storage validation, providing enterprise customers and channel partners with flexible storage reference architectures, validated against key benchmarks to provide a strong infrastructure foundation for cost- and performance-optimised AI hardware and software solutions. This validation offers additional choice for AI customers and complements Pure Storage’s certification for NVIDIA DGX BasePOD announced last year.
- Vertical RAG Development. To accelerate successful AI adoption across vertical industries, Pure Storage is creating vertical-specific RAGs in collaboration with NVIDIA. First, Pure Storage has created a financial services RAG solution to summarise and query massive datasets with higher accuracy than off-the-shelf LLMs. Financial services institutions can now gain faster insight using AI to create instant summaries and analysis from various financial documents and other sources. Additional RAGs for healthcare and public sector are to be released.
- Expanded Investment in AI Partner Ecosystem. Pure Storage is further investing in its AI partner ecosystem with NVIDIA, engaging in new partnerships with ISVs like Run.AI and Weights & Biases. While Run.AI optimises GPU utilisation through advanced orchestration and scheduling, the Weights & Biases AI Development platform enables ML teams to build, evaluate, and govern the model development lifecycle. Additionally, Pure Storage is working closely with AI-focused reseller and service partners including ePlus, Insight, WWT, and others to further operationalize joint customer AI deployments.
“At World Wide Technology, technology innovation is at the core of delivering valuable services to our global clients. As the demand for AI grows, we couldn’t be more excited to continue partnering with two best-of-breed vendors in our AI Proving Ground. Pure Storage’s high-performance AI storage infrastructure and NVIDIA’s prowess in AI computing creates an impressive synergy, enabling our joint customers to seamlessly infuse artificial intelligence into their business operations while driving meaningful ROI,” said Ryan Avery, High-Performance Storage Architect at World Wide Technology.
Industry Significance
Today, the majority of AI deployments are dispersed across fragmented data environments — from the cloud to ill-suited (often legacy) storage solutions. Yet these fragmented environments cannot support the performance and networking requirements to fuel AI data pipelines and unlock the full potential of enterprise data.
As enterprises further embrace AI to drive innovation, streamline operations, and gain a competitive edge, the demand for robust, high-performance, and efficient AI infrastructure has never been stronger.
“Our mission at Run.AI is to be a driving force in the AI revolution, leading the charge for positive change across industries and enhancing the human experience. Focusing our efforts on providing the essential software layer that empowers AI infrastructure, we are thrilled to partner with Pure Storage and NVIDIA to help drive broader (and more successful) adoption of advanced enterprise AI initiatives,” noted Yael Dor, VP, Global Sales and Partnerships at Run.AI.
Pioneering enterprise AI deployments, particularly among a rapidly growing set of Fortune 500 enterprise customers, Pure Storage provides a simple, reliable, and efficient storage platform for enterprises to fully leverage the potential of AI, while reducing the associated risk, cost, and energy consumption.
“Pure Storage’s NVIDIA-validated reference architectures and proofs of concept provide enterprises across industries a shortcut to AI success. Rather than investing valuable time and resources in building an AI architecture from scratch, Pure’s proven frameworks not only mitigate the risk of expensive project delays but also guarantee a high return on investment for AI team expenditures like GPUs,” added Mike Leone, Principal Analyst at ESG.


Archive
- October 2024(44)
- September 2024(94)
- August 2024(100)
- July 2024(99)
- June 2024(126)
- May 2024(155)
- April 2024(123)
- March 2024(112)
- February 2024(109)
- January 2024(95)
- December 2023(56)
- November 2023(86)
- October 2023(97)
- September 2023(89)
- August 2023(101)
- July 2023(104)
- June 2023(113)
- May 2023(103)
- April 2023(93)
- March 2023(129)
- February 2023(77)
- January 2023(91)
- December 2022(90)
- November 2022(125)
- October 2022(117)
- September 2022(137)
- August 2022(119)
- July 2022(99)
- June 2022(128)
- May 2022(112)
- April 2022(108)
- March 2022(121)
- February 2022(93)
- January 2022(110)
- December 2021(92)
- November 2021(107)
- October 2021(101)
- September 2021(81)
- August 2021(74)
- July 2021(78)
- June 2021(92)
- May 2021(67)
- April 2021(79)
- March 2021(79)
- February 2021(58)
- January 2021(55)
- December 2020(56)
- November 2020(59)
- October 2020(78)
- September 2020(72)
- August 2020(64)
- July 2020(71)
- June 2020(74)
- May 2020(50)
- April 2020(71)
- March 2020(71)
- February 2020(58)
- January 2020(62)
- December 2019(57)
- November 2019(64)
- October 2019(25)
- September 2019(24)
- August 2019(14)
- July 2019(23)
- June 2019(54)
- May 2019(82)
- April 2019(76)
- March 2019(71)
- February 2019(67)
- January 2019(75)
- December 2018(44)
- November 2018(47)
- October 2018(74)
- September 2018(54)
- August 2018(61)
- July 2018(72)
- June 2018(62)
- May 2018(62)
- April 2018(73)
- March 2018(76)
- February 2018(8)
- January 2018(7)
- December 2017(6)
- November 2017(8)
- October 2017(3)
- September 2017(4)
- August 2017(4)
- July 2017(2)
- June 2017(5)
- May 2017(6)
- April 2017(11)
- March 2017(8)
- February 2017(16)
- January 2017(10)
- December 2016(12)
- November 2016(20)
- October 2016(7)
- September 2016(102)
- August 2016(168)
- July 2016(141)
- June 2016(149)
- May 2016(117)
- April 2016(59)
- March 2016(85)
- February 2016(153)
- December 2015(150)