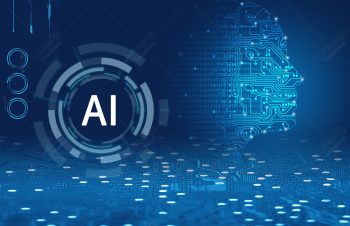
In a research report commissioned by Hewlett Packard Enterprise (HPE) titled “Architect an AI Advantage,” nearly half (44%) of IT leaders surveyed believe their organisations are fully set up to realise the benefits of AI. However, the report reveals critical gaps in their strategies, such as lack of alignment between processes and metrics, resulting in consequential fragmentation in approach, which will further exacerbate delivery issues.
The “Architect an AI Advantage” report, which surveyed more than 2,000 IT leaders from 14 countries, found that while global commitment to Artificial Intelligence (AI) shows growing investments, businesses are overlooking key areas that will have a bearing on their ability to deliver successful AI outcomes—including low data maturity levels, possible deficiencies in their networking and compute provisioning, and vital ethics and compliance considerations.
The “Architect an AI Advantage” report also uncovered significant disconnects in both strategy and understanding that could adversely affect future return on investment (ROI).
“Enterprises in APAC are understandably eager to embrace AI to reap its many transformative benefits,” said Joseph Yang, General Manager, HPC and AI, APAC and India, at HPE. “Yet, as the findings from our survey clearly indicate, many organisations are not yet ready for effective and safe AI deployments. We are seeing critical blind spots in their AI strategies, including overlooking ethics and compliance, that could lead to serious consequences on the business. As AI investments continue to soar, it’s important that organisations devise a holistic AI roadmap that addresses these blind spots to ensure AI success and optimise their ROI.”
“Architect an AI Advantage” Report Uncovers Low Data Maturity
Strong AI performance that impacts business outcomes depends on quality data input, but the research shows that while organisations clearly understand this—labelling data management as one of the most critical elements for AI success—their data maturity levels remain low.
According to the “Architect an AI Advantage” report, only a small percentage (7%) of organisations can run real-time data pushes/pulls to enable innovation and external data monetisation, while just 26% have set up data governance models and can run advanced analytics.
Of greater concern, fewer than 6 in 10 respondents said their organisation is completely capable of handling any of the key stages of data preparation for use in AI models—from accessing (59%) and storing (57%) to processing (55%) and recovering (51%). This discrepancy not only risks slowing down the AI model creation process, but also increases the probability the model will deliver inaccurate insights and a negative ROI.
Provisioning for the End-to-End Lifecycle
A similar gap appeared when respondents were asked about the compute and networking requirements across the end-to-end AI lifecycle.
On the surface, confidence levels look high in this regard: 93% of IT leaders believe their network infrastructure is set up to support AI traffic, while 84% agree their systems have enough flexibility in compute capacity to support the unique demands across different stages of the AI lifecycle.
Gartner® expects that “GenAI will play a role in 70% of text- and data-heavy tasks by 2025, up from less than 10% in 2023,” yet less than half of IT leaders admitted to having a full understanding of what the demands of the various AI workloads across training, tuning and inferencing might be—calling into serious question how accurately they can provision for them.
Ignoring Cross-Business Connections, Compliance, and Ethics
Organisations, according to the “Architect an AI Advantage” report, are failing to connect the dots between key areas of business, with over a quarter (28%) of IT leaders describing their organisation’s overall AI approach as “fragmented.” As evidence of this, over a third (35%) of organisations have chosen to create separate AI strategies for individual functions, while 32% are creating different sets of goals altogether.
More dangerous still, it appears that ethics and compliance are being completely overlooked, despite growing scrutiny around ethics and compliance from both consumers and regulatory bodies. The “Architect an AI Advantage” report shows that legal/compliance (13%) and ethics (11%) were deemed by IT leaders to be the least critical for AI success.
In addition, the results of the “Architect an AI Advantage” report showed that almost 1 in 4 organisations (22%) are not involving legal teams in their business’s AI strategy conversations at all.
The Fear of Missing Out on AI and the Business Risk of Overconfidence
As businesses move quickly to understand the hype around AI, without proper AI ethics and compliance, businesses run the risk of exposing their proprietary data—a cornerstone for retaining their competitive edge and maintaining their brand reputation.
Among the issues, businesses lacking an AI ethics policy risk developing models that lack proper compliance and diversity standards, resulting in negative impacts to the company’s brand, loss in sales or costly fines and legal battles. There are additional risks as well, as the quality of the outcomes from AI models is limited to the quality of the data they ingest.
This is reflected in the “Architect an AI Advantage” report, which shows data maturity levels remain low. When combined with the metric that half of IT leaders admitted to having a lack of full understanding of the IT infrastructure demands across the AI lifecycle, there is an increase in the overall risk of developing ineffective models, including the impact from AI hallucinations.
Additionally, as the power demand to run AI models is extremely high, this can contribute to an unnecessary increase in data centre carbon emissions. These challenges lower the ROI from a company’s capital investment in AI and can further negatively impact the overall company brand.
“AI is the most data and power-intensive workload of our time, and to effectively deliver on the promise of GenAI, solutions must be hybrid by design and built with a modern AI architecture,” said Dr Eng Lim Goh, SVP for Data & AI, at HPE. “From training and tuning models on-premises, in a colocation or in the public cloud, to inferencing at the edge, GenAI has the potential to turn data into insights from every device on the network. However, businesses must carefully weigh the balance of being a first mover, and the risk of not fully understanding the gaps across the AI lifecycle, otherwise the large capital investments can end up delivering a negative ROI.”


Archive
- October 2024(44)
- September 2024(94)
- August 2024(100)
- July 2024(99)
- June 2024(126)
- May 2024(155)
- April 2024(123)
- March 2024(112)
- February 2024(109)
- January 2024(95)
- December 2023(56)
- November 2023(86)
- October 2023(97)
- September 2023(89)
- August 2023(101)
- July 2023(104)
- June 2023(113)
- May 2023(103)
- April 2023(93)
- March 2023(129)
- February 2023(77)
- January 2023(91)
- December 2022(90)
- November 2022(125)
- October 2022(117)
- September 2022(137)
- August 2022(119)
- July 2022(99)
- June 2022(128)
- May 2022(112)
- April 2022(108)
- March 2022(121)
- February 2022(93)
- January 2022(110)
- December 2021(92)
- November 2021(107)
- October 2021(101)
- September 2021(81)
- August 2021(74)
- July 2021(78)
- June 2021(92)
- May 2021(67)
- April 2021(79)
- March 2021(79)
- February 2021(58)
- January 2021(55)
- December 2020(56)
- November 2020(59)
- October 2020(78)
- September 2020(72)
- August 2020(64)
- July 2020(71)
- June 2020(74)
- May 2020(50)
- April 2020(71)
- March 2020(71)
- February 2020(58)
- January 2020(62)
- December 2019(57)
- November 2019(64)
- October 2019(25)
- September 2019(24)
- August 2019(14)
- July 2019(23)
- June 2019(54)
- May 2019(82)
- April 2019(76)
- March 2019(71)
- February 2019(67)
- January 2019(75)
- December 2018(44)
- November 2018(47)
- October 2018(74)
- September 2018(54)
- August 2018(61)
- July 2018(72)
- June 2018(62)
- May 2018(62)
- April 2018(73)
- March 2018(76)
- February 2018(8)
- January 2018(7)
- December 2017(6)
- November 2017(8)
- October 2017(3)
- September 2017(4)
- August 2017(4)
- July 2017(2)
- June 2017(5)
- May 2017(6)
- April 2017(11)
- March 2017(8)
- February 2017(16)
- January 2017(10)
- December 2016(12)
- November 2016(20)
- October 2016(7)
- September 2016(102)
- August 2016(168)
- July 2016(141)
- June 2016(149)
- May 2016(117)
- April 2016(59)
- March 2016(85)
- February 2016(153)
- December 2015(150)