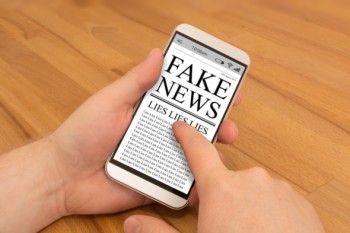
The same techniques used to identify and motivate likely voters can also be used to spread false information
Joseph Goebbels said “If you tell a lie big enough and keep repeating it, people will eventually come to believe it.” In the era of big data, however, numerous smaller lies, guided by machine learning, may be more effective than a few big lies. And even the literal truth seasoned with innuendo will do. Studies show that the salient concept in a statement can persist and dominate the literal truth in which it is embedded. For example, consider the statement, “There is no evidence that Hillary Clinton ran a child sex slavery operation out of Comet Pizza.” Repeat it often enough, and people are prone to remember just the “Hillary Clinton… child sex slavery” concept, not its negation.
President Trump’s disregard for the truth often seems impulsive, and not strategic—a bit like that of a braggart in a bar (were it not for the fact that he is a teetotaler). Reacting angrily to news reports that his inauguration crowd was not as big as that of President Obama when he first came into office, or even as big as the protesting crowd gathered by the Women’s March, Trump insisted (and had his press secretary insist) that his inaugural crowds were the biggest in U.S. history (not true). During the campaign, he claimed to have seen people in Jersey City celebrating the 9/11 attack by dancing in the street (also not true). After the election, he claimed that millions of votes by illegal immigrants cost him the popular vote (no evidence).
President Trump’s enthusiastic embrace of casual lying is partly seen as a reflection and a product of the general phenomenon of “fake news.” The impact of fake news is real—Comet Pizza, a restaurant in Washington DC, was indeed believed by many to be the site of a child sex trafficking ring that was operating with the involvement of Hillary Clinton. It was the scene of a bizarre siege on Dec. 4 when Edgar Welch, heavily armed, entered the restaurant and announced that he was there to investigate matters. The sex-slave story was pure fiction, but it was all over the internet. Edgar Welch believed it, and traveled to DC from North Carolina, ready to do battle.
Where does fake news come from? It turns out there is a profitable cottage industry in creating and purveying fake news. NPR tracked down one fake-news creator, Jestin Coler, in a Los Angeles suburb. Coler started out catering to the alt-right, and found a rapidly expanding audience during the recent presidential campaign—“a huge Facebook group of kind of rabid Trump supporters just waiting to eat up this red meat.”
Jestin Coler is a Democrat, and says he wants to expose the phenomenon of fake news. But the money is good (over $100,000 per year), and, in the end, what he wanted people to do was to click on his stories so he could collect advertising revenue.
But suppose you were interested in getting someone to do something more than click? Something political—like vote for a particular candidate, go to a rally, write your Congressman, etc.? That’s where Big Data comes into the picture.
Since 2004, political consultants have used big-data models to predict how people will vote, and indicate whether they should be sent messages to encourage them to do so (and if so, which messages). They use randomized experiments (A-B tests) to determine the effect of different messages at the individual level, and correlate this with other variables, such as demographic data and voting data, to build predictive models. All this is similar to what happens in the marketing realm (e.g. should a given consumer be sent solicitation A or B), and President Obama was a pioneer in the use of predictive analytics to target individual voters.
The science of predictive modeling has come a long way since 2004. Statisticians now build “personality” models and tie them into other predictor variables. Edgar Welch can now be targeted for messaging not simply on the basis of his demographic voting behavior, but on the basis of a personality classification derived from reams of detailed personal data available for purchase. One such model bears the acronym “OCEAN,” standing for the personality characteristics (and their opposites) of openness, conscientiousness, extroversion, agreeableness, and neuroticism. Using Big Data at the individual level, machine learning methods might classify a person as, for example, “closed, introverted, neurotic, not agreeable, and conscientious.”
Alexander Nix, CEO of Cambridge Analytica (owned by Trump’s chief donor, Rebekah Mercer), says he has thousands of data points on you, and every other voter: what you buy or borrow, where you live, what you subscribe to, what you post on social media, etc. At a recent Concordia Summit, using the example of gun rights, Nix described how messages will be crafted to appeal specifically to you, based on your personality profile. Are you highly neurotic and conscientious? Nix suggests the image of a sinister gloved hand reaching through a broken window.
In his presentation, Nix noted that the goal is to induce behavior, not communicate ideas. So where does truth fit in? Johan Ugander, Assistant Professor of Management Science at Stanford, suggests that, for Nix and Cambridge Analytica, it doesn’t. In counseling the hypothetical owner of a private beach how to keep people off his property, Nix eschews the merely factual “Private Beach” sign, advocating instead a lie: “Sharks sighted.” Ugander, in his critique, cautions all data scientists against “building tools for unscrupulous targeting.”
The warning is needed, but may be too late. What Nix described in his presentation involved carefully crafted messages aimed at his target personalities. His messages pulled subtly on various psychological strings to manipulate us, and they obeyed no boundary of truth, but they required humans to create them. The next phase will be the gradual replacement of human “craftsmanship” with machine learning algorithms that can supply targeted voters with a steady stream of content (from whatever source, true or false) designed to elicit desired behavior. Cognizant of the Pandora’s box that data scientists have opened, the scholarly journal Big Data has issued a call for papers for a future issue devoted to “Computational Propaganda.”
Hopefully, it will address broader ethical and policy issues, and not be a “how to” manual.
This article was originally published on www.blogs.scientificamerican.com and can be viewed in full


Archive
- October 2024(44)
- September 2024(94)
- August 2024(100)
- July 2024(99)
- June 2024(126)
- May 2024(155)
- April 2024(123)
- March 2024(112)
- February 2024(109)
- January 2024(95)
- December 2023(56)
- November 2023(86)
- October 2023(97)
- September 2023(89)
- August 2023(101)
- July 2023(104)
- June 2023(113)
- May 2023(103)
- April 2023(93)
- March 2023(129)
- February 2023(77)
- January 2023(91)
- December 2022(90)
- November 2022(125)
- October 2022(117)
- September 2022(137)
- August 2022(119)
- July 2022(99)
- June 2022(128)
- May 2022(112)
- April 2022(108)
- March 2022(121)
- February 2022(93)
- January 2022(110)
- December 2021(92)
- November 2021(107)
- October 2021(101)
- September 2021(81)
- August 2021(74)
- July 2021(78)
- June 2021(92)
- May 2021(67)
- April 2021(79)
- March 2021(79)
- February 2021(58)
- January 2021(55)
- December 2020(56)
- November 2020(59)
- October 2020(78)
- September 2020(72)
- August 2020(64)
- July 2020(71)
- June 2020(74)
- May 2020(50)
- April 2020(71)
- March 2020(71)
- February 2020(58)
- January 2020(62)
- December 2019(57)
- November 2019(64)
- October 2019(25)
- September 2019(24)
- August 2019(14)
- July 2019(23)
- June 2019(54)
- May 2019(82)
- April 2019(76)
- March 2019(71)
- February 2019(67)
- January 2019(75)
- December 2018(44)
- November 2018(47)
- October 2018(74)
- September 2018(54)
- August 2018(61)
- July 2018(72)
- June 2018(62)
- May 2018(62)
- April 2018(73)
- March 2018(76)
- February 2018(8)
- January 2018(7)
- December 2017(6)
- November 2017(8)
- October 2017(3)
- September 2017(4)
- August 2017(4)
- July 2017(2)
- June 2017(5)
- May 2017(6)
- April 2017(11)
- March 2017(8)
- February 2017(16)
- January 2017(10)
- December 2016(12)
- November 2016(20)
- October 2016(7)
- September 2016(102)
- August 2016(168)
- July 2016(141)
- June 2016(149)
- May 2016(117)
- April 2016(59)
- March 2016(85)
- February 2016(153)
- December 2015(150)