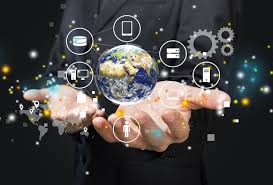
Not much of consequence happens without risk. As more organizations realize the value of Hadoop while they look to adopt big data into their technology portfolio, they also need to consider the inherent potential for negative consequences. Big data has opened up a whole new world of risk, but that’s not stopping — or even slowing — many businesses looking to cash in on the rewards. To balance this process, technology and business leaders should know how to manage the conversations around big data risks as well as rewards.
When viewed through the lens of risk, organizations have different classifications and considerations to own:
1. Data security and administration
Data security and administration are the obvious issues that usually get the first look. But there are many technical layers for appreciating the security of your data, including:
- perimeter security
- data encrypted at rest and in transit
- proper configuration for authentication, provisioning, onboarding, offboarding
- high availability and failover
- bare metal versus cloud
Who is going to manage this environment? Can you find the talent to stand up, lock down and maintain your big data stack? When new big data initiatives are launched, these questions are the first things that IT and your information security team will want to know. Be ready with the answers, and know why these things are important for securing funding and buy-in.
2. Data governance
How do you manage the ingress and organization of the data? One of the hidden risks of a comprehensive data lake is that data from one source can be combined with data from another to create inadvertent data exposures. The unforeseen downside of bringing in all the data to one place is that existing controls and processes for privacy may be obviated. Data governance is more than that, of course, but be ready to have a data governance strategy, embrace it and partner with your data stewards early in the process.
3. Speed-to-market philosophy
Is there a cost to NOT having the tools in place, like not being able to leverage your data assets? This is a new technology landscape – business analysts have to learn how to hunt for their own data. The onus for coding business rules into viable code has shifted responsibilities from process-heavy IT functions to results-oriented business units. With great power comes great responsibility, but you should trust your people and reward them with your “data first” ethos.
4. Are you just recreating existing processes with different technologies?
This is one of the biggest latent risks because it indicates that the technologies have evolved but your mindset has not. It can be like using a hammer to drive in a screw. You just spent a lot of money to recreate your data warehouse in Hadoop – and that’s not what it’s for. Understanding the differences between a data lake and a data warehouse will be important, and be ready to preach this on a daily basis.
5. What if this is all a fad and the traditional vendors catch up?
There are vendor management implications, for sure. Maybe it would just be easier on procurement if a database just released their own big data stack? Unfortunately, that’s not how this works. Organizations need to accept that big data environments are complements to their existing technology stack, and that the new players are approaching data analytics from a different perspective.
The Big Picture
Organizations need to understand – if not obsess about — the relationships between their big data environment and the inherent risks associated with having or not having one. Innovation will not arrive without risk, and when thoughtfully managed and understood, your organization will be better prepared to move forward.
The rewards and bounty for succeeding with big data are just now being realized. For some organizations, that means better customer service, retention or acquisition. Profits may improve by creating new, sophisticated product recommendations. For other organizations, fraud identification and prevention techniques are reducing overall costs and isolating additional risk points. All kinds of big data risk/benefit scenarios are emerging, and many companies have concluded that they are ready because they took the time to weigh the risks and convey the “whys” throughout their company. Because if you can’t assess the yield from your big data strategy, you aren’t ready to take that first, risky Big step.
This article was originally published on www.datamation.com and can be viewed in full


Archive
- October 2024(44)
- September 2024(94)
- August 2024(100)
- July 2024(99)
- June 2024(126)
- May 2024(155)
- April 2024(123)
- March 2024(112)
- February 2024(109)
- January 2024(95)
- December 2023(56)
- November 2023(86)
- October 2023(97)
- September 2023(89)
- August 2023(101)
- July 2023(104)
- June 2023(113)
- May 2023(103)
- April 2023(93)
- March 2023(129)
- February 2023(77)
- January 2023(91)
- December 2022(90)
- November 2022(125)
- October 2022(117)
- September 2022(137)
- August 2022(119)
- July 2022(99)
- June 2022(128)
- May 2022(112)
- April 2022(108)
- March 2022(121)
- February 2022(93)
- January 2022(110)
- December 2021(92)
- November 2021(107)
- October 2021(101)
- September 2021(81)
- August 2021(74)
- July 2021(78)
- June 2021(92)
- May 2021(67)
- April 2021(79)
- March 2021(79)
- February 2021(58)
- January 2021(55)
- December 2020(56)
- November 2020(59)
- October 2020(78)
- September 2020(72)
- August 2020(64)
- July 2020(71)
- June 2020(74)
- May 2020(50)
- April 2020(71)
- March 2020(71)
- February 2020(58)
- January 2020(62)
- December 2019(57)
- November 2019(64)
- October 2019(25)
- September 2019(24)
- August 2019(14)
- July 2019(23)
- June 2019(54)
- May 2019(82)
- April 2019(76)
- March 2019(71)
- February 2019(67)
- January 2019(75)
- December 2018(44)
- November 2018(47)
- October 2018(74)
- September 2018(54)
- August 2018(61)
- July 2018(72)
- June 2018(62)
- May 2018(62)
- April 2018(73)
- March 2018(76)
- February 2018(8)
- January 2018(7)
- December 2017(6)
- November 2017(8)
- October 2017(3)
- September 2017(4)
- August 2017(4)
- July 2017(2)
- June 2017(5)
- May 2017(6)
- April 2017(11)
- March 2017(8)
- February 2017(16)
- January 2017(10)
- December 2016(12)
- November 2016(20)
- October 2016(7)
- September 2016(102)
- August 2016(168)
- July 2016(141)
- June 2016(149)
- May 2016(117)
- April 2016(59)
- March 2016(85)
- February 2016(153)
- December 2015(150)