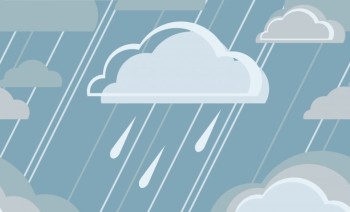
Enterprises have been slow to move big data processing to the cloud, but not for lack of trying.
Most companies now use the public cloud in some form, often for SaaS applications. But enterprises have been slow to migrate big data and data warehousing to the cloud, despite cost, scalability and elasticity benefits. According to a 2014 Gartner survey, less than half of organizations with big data programs reported using the cloud in any form.
This raises the question – why aren’t more companies using the cloud for data processing, especially since concerns over security are easing and more services are available than ever before?
There’s a simple answer: It’s challenging – especially for enterprises.
To paraphrase investor Ben Horowitz, “It’s not checkers – it’s … chess.”
Much of it depends on how your infrastructure grew up. Many digital natives, with their businesses entirely online, built cloud-based systems from the get-go.
But for established enterprises with significant investments in on-premise data centers, using the cloud requires that they learn, maintain, and integrate two environments – technically, culturally, and operationally.
One CIO recently shared more with a memorable analogy: “It took us about eight months to create a new data warehousing environment in the cloud. We hired someone, sent a few people to training, and brought in consultants. Now it’s live, but it’s like accessing a space station. Getting data up there and using it requires a significant effort – a major mission every time. So, cloud is not part of our normal processes and it’s not saving us money yet. We’re barely using it.”
At Cazena, we hear stories like this all the time.
IT leaders report they’re often surprised where the biggest cloud challenges have cropped up. The good news is that none of these issues are insurmountable, especially if you plan for them and choose the right services for your requirements.
Here are six challenges of big data in the cloud and how to overcome them.
Clouds are from Venus, data centers are from Mars
Like men and women, cloud services and on-premises data centers are vastly different worlds – a fact often underestimated by enterprises.
Cloud services are set up, configured, and priced differently than standard servers in a data center. To start, budgeting and managing costs changes completely under the rental paradigm of the cloud versus the traditional model. Choosing and optimizing cloud infrastructure for big data workloads requires new skills that must be learned or acquired.
While it may be easy for a developer to spin up a quick experiment, it is notoriously challenging to integrate cloud services with existing systems. And that’s just setting up basic services – never mind architecture, deployment, integration, and managing the cloud.
Deciding the right skills to source is hard
“Lack of resources/ expertise” is now the number one challenge in cloud, edging out security in the 2016 RightScale: State of the Cloud Survey.
Experts cite major shortages in cloud architecture, developer skills, and cloud DevOps.
Related is the well-documented big data skills shortage. Big data and cloud expertise is difficult – and expensive – to find.
Getting from experimentation to production is a big leap
Many enterprises report that they have piloted the cloud for big data. But as with the weary CIO above and his “space station,” companies say they find it hard to make the cloud an integrated part of production data processes.
In addition to the complex data and system integration work, adding a new cloud environment also brings change management and operational hurdles. Enterprises report it’s more difficult for them to determine and uphold service-level agreements (SLAs) for cloud services.
How can two-second response times for business intelligent queries be delivered most cost-effectively, versus overnight batch processes? Overall, it’s a major challenge to support the cloud as they do other services.
Large enterprises that have successfully developed their own cloud analytics capabilities typically invest several years and millions of dollars in the effort.
Cloud security is widely misunderstood
Historically, security and privacy topped surveys of cloud challenges, but experts now say cloud providers are more secure than ever before.
Problems often result from inconsistent, ad hoc use of the cloud, or poorly-defined cloud policies, leading to human mistakes. Gartner predicts that through 2020, 95 percent of cloud security failures will be the customer’s fault. The same report goes on to point out that companies should not be scared off by this statistic.
On the contrary, enterprises simply need to formally address cloud security, leaving no room for interpretation. Companies must also learn to correctly use the security features offered by cloud providers, and choose vendors that can work with their existing management systems and policies.
Data movement is super challenging
The emphasis is also misplaced in the well-covered issue of moving data into the cloud. Many discussions focus on how giant petabyte-sized datasets move from data centers into the cloud.
In fact, significant volumes of data like this are usually moved in physical media like Amazon Snowball or by FedEx or other ground transportation. But these are often one-time, massive transfers, not ongoing updates.
The real challenge is making data movement to and from the cloud a seamless part of the enterprise data flow. Discussions about data movement to the cloud must focus on streaming, micro-batching updates, and data pipelines. For the cloud to make an impact on production processes, enterprises must consider ongoing, two-way data movement.
Many companies want to use the cloud to collect and pre-process data, then move subsets to on-premises data warehouses. It’s not just about moving data from data centers into the cloud.
Cloud services are not yet standardized
Emerging tech markets often produce wildly varying labels, capabilities, and pricing models. This is especially true for data processing in the cloud, where buyers must dig deep to understand the differences between numerous point solutions for specific technologies and broader managed service offerings.
Some services are pay-as-you-go; some are flat-rate. They include different levels of monitoring, management, and SLAs. And cloud vendors vary in their levels of operational support.
The big challenge is finding cloud providers that can fit comfortably with your existing processes.
None of these challenges warrant staying out of the cloud. With its processing power, scale and economics, cloud is the the future of big data analytics. As savvy digital natives have proven, using the cloud for analytics enables a major competitive edge.
Perhaps your biggest resource is the plethora of value-added services available today.
The cloud is no longer a DIY environment for developers. While enterprises have been slow to move to the cloud, vendors have been busy developing new services to ease the transition.
Now, it’s key to pick cloud projects carefully, choosing well-scoped endeavors that augment your overall capabilities. Find the right balance of the cloud services you source versus strategic skills you develop in-house.
Here are two best practices:
Hone your cloud service provider evaluation skills
Never assume any consistency across services. Evaluate each carefully with a similar requirements matrix, and use industry expertise to make sure you cover all your options.
With each service, examine and test integration capabilities upfront to determine how the solution will fit into your existing architecture.
Understand exactly what you’re getting from your cloud provider for security, and delineate roles and responsibilities for cloud management.
Determine which strategic skills to hire or develop
New services and solutions are rapidly filling the gaps between cloud and enterprise. Managed services supplement enterprise resources, while “big data as a service” offerings abstract and automate underlying cloud complexity.
These services provide alternatives to building a custom cloud data platform from scratch, allowing you to focus your resources on data strategy and data science to interpret and use data. Focus on developing the in-house skills that give you a sustainable edge.
Armed with these principles, you can successfully move big data processing to the cloud.


Archive
- October 2024(44)
- September 2024(94)
- August 2024(100)
- July 2024(99)
- June 2024(126)
- May 2024(155)
- April 2024(123)
- March 2024(112)
- February 2024(109)
- January 2024(95)
- December 2023(56)
- November 2023(86)
- October 2023(97)
- September 2023(89)
- August 2023(101)
- July 2023(104)
- June 2023(113)
- May 2023(103)
- April 2023(93)
- March 2023(129)
- February 2023(77)
- January 2023(91)
- December 2022(90)
- November 2022(125)
- October 2022(117)
- September 2022(137)
- August 2022(119)
- July 2022(99)
- June 2022(128)
- May 2022(112)
- April 2022(108)
- March 2022(121)
- February 2022(93)
- January 2022(110)
- December 2021(92)
- November 2021(107)
- October 2021(101)
- September 2021(81)
- August 2021(74)
- July 2021(78)
- June 2021(92)
- May 2021(67)
- April 2021(79)
- March 2021(79)
- February 2021(58)
- January 2021(55)
- December 2020(56)
- November 2020(59)
- October 2020(78)
- September 2020(72)
- August 2020(64)
- July 2020(71)
- June 2020(74)
- May 2020(50)
- April 2020(71)
- March 2020(71)
- February 2020(58)
- January 2020(62)
- December 2019(57)
- November 2019(64)
- October 2019(25)
- September 2019(24)
- August 2019(14)
- July 2019(23)
- June 2019(54)
- May 2019(82)
- April 2019(76)
- March 2019(71)
- February 2019(67)
- January 2019(75)
- December 2018(44)
- November 2018(47)
- October 2018(74)
- September 2018(54)
- August 2018(61)
- July 2018(72)
- June 2018(62)
- May 2018(62)
- April 2018(73)
- March 2018(76)
- February 2018(8)
- January 2018(7)
- December 2017(6)
- November 2017(8)
- October 2017(3)
- September 2017(4)
- August 2017(4)
- July 2017(2)
- June 2017(5)
- May 2017(6)
- April 2017(11)
- March 2017(8)
- February 2017(16)
- January 2017(10)
- December 2016(12)
- November 2016(20)
- October 2016(7)
- September 2016(102)
- August 2016(168)
- July 2016(141)
- June 2016(149)
- May 2016(117)
- April 2016(59)
- March 2016(85)
- February 2016(153)
- December 2015(150)