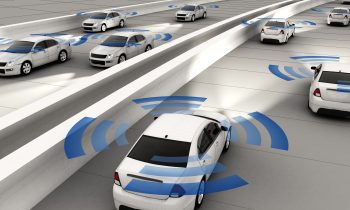
Written By: Hwee Yng Yeo, Smart Mobility Advocate, Keysight Technologies
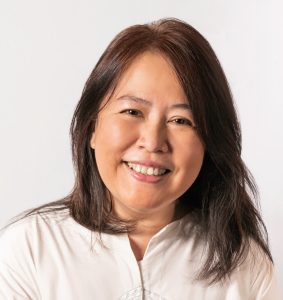
Hwee Yng Yeo, Smart Mobility Advocate, Keysight Technologies
Automotive radar systems need more rigorous testing as they assume critical functions in many of today’s Currently, automakers and radar module providers test the functionality of their radar modules using both software and hardware. There are two key methods for hardware-based tests.
The first uses corner reflectors that are placed at different distances and angles from the radar device under test (DUT), with each reflector representing a static target. When a change of this static scenario is needed, the corner reflectors must be physically moved to their new positions. The procedures are time-consuming and add to overall test time. Each movement of the antennas introduces a change in the echo’s angle of arrival, which might lead to errors and loss of accuracy in rendering targets if not recalculated or recalibrated.
The second uses a radar target simulator (RTS) that enables an electronic simulation of the radar targets, thus allowing for both static and dynamic targets, along with simulating the target’s distance, velocity, and size. Limitations of RTS-based functional testing arise for complex / realistic scenarios with more than 32 targets. RTS-based testing also cannot characterize 4D (including vertical positions, on top of distance, horizontal positions, and velocities) and imaging radar capabilities for detecting extended objects, which are objects represented by point clouds instead of just one reflection.
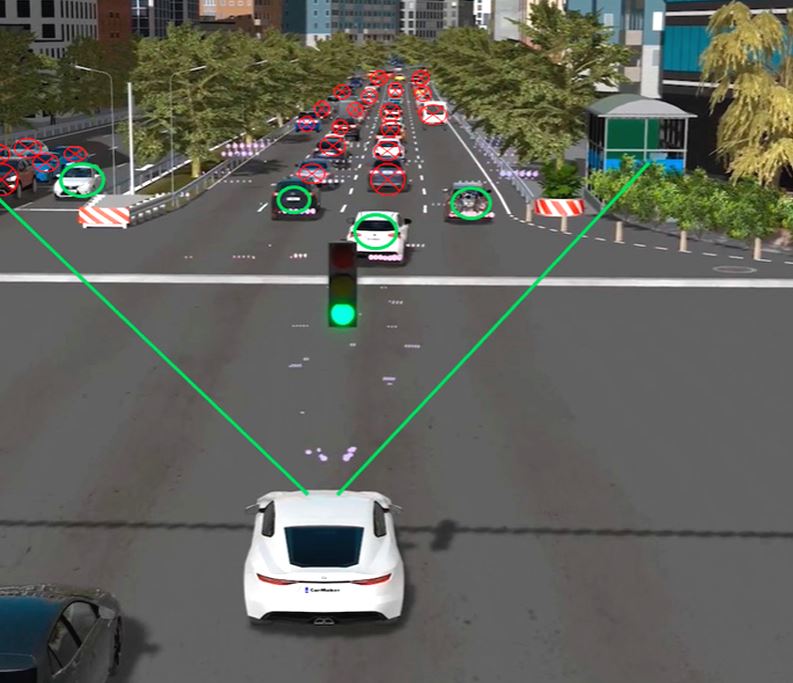
Figure 1. Testing automotive radar sensors with radar target simulation does not provide a complete traffic scene for validating autonomous driving applications.
Testing radar units against a limited number of objects delivers an incomplete view of driving scenarios for the AV. It masks the complexity of the real world, especially in urban areas with different intersections and turning scenarios involving pedestrians, cyclists, and electric scooters.
Smartening Automotive Radar Algorithms
Increasingly, machine learning is helping developers train their ADAS algorithms to better interpret and classify data from radar sensors and other sensor systems. More recently, the term “YOLO” has surfaced amongst headlines on automotive radar algorithms. The acronym stands for “You only look once.” This acronym is apt, given what the radar perceives and how the ADAS algorithms interpret the data are mission-critical processes, which can be a matter of life or death. YOLO-based radar target detection aims to accurately detect and classify multiple objects simultaneously.
It is crucial to subject both the physical radar sensors and the ADAS algorithms to rigorous testing before these self-driving systems move onto the final costly road-testing stage. To create a more realistic 360o view of varying real-world traffic scenarios, automakers have started using radar scene emulation technology to bring the road to the lab.
One of the key challenges of upgrading autonomous driving is the ability to distinguish between dynamic obstacles on the road and autonomously deciding on the course of action versus just raising a flag or flashing a warning on the dashboard. In emulating a traffic scenario, too few points per object may cause a radar to erroneously detect closely spaced targets as a single entity. This makes it difficult to fully test not just the sensor but also the algorithms and decisions that rely on data streaming from the radar sensor.
A new radar scene emulation technology uses ray tracing and point clouds to extract the relevant data from highly realistic simulated traffic scenes and provides better detection and differentiation of objects (see Figure 2). Using novel millimetre wave (mmWave) over-the-air technology, the radar scene emulator can generate multiple static and dynamic targets from as close as 1.5 metres to as far away as 300 meters, and with velocities of 0 to 400 km/h for short, medium, and long-range automotive radars. This provides a much more realistic traffic scenario against which the automotive radar sensors can be tested.

Figure 2. A screen capture of perception algorithm testing using radar scene emulation. The right screen shows a simulated traffic scenario which is emulated by the radar scene emulator on the left. The green dots represent emulated radar reflections while the red dots show radar sensor detections.
Radar scene emulation is extremely useful for pre-roadway drive tests as both automotive radar sensors and the algorithms can undergo numerous design iterations quickly to fix bugs and finetune designs. Apart from ADAS and autonomous driving functional testing, it can help automakers with variant handling applications, such as validating the effect of different bumper designs, paintwork, and radar module positioning on radar functions.
For autonomous driving platform providers and radar systems manufacturers, enhancing the vehicle’s perception of different realistic traffic scenes through multiple repeatable and customisable scenarios can allow the radar sensors to capture vast amounts of data for Machine Learning by autonomous driving algorithms.
Nowadays, advanced digital signal processing (DSP) also plays a crucial role in enabling finetuning of individual radar detections. For instance, the radar can pick up different points of a pedestrian’s arms and legs, including the velocity, distance, cross section (size), and angle (both horizontally and vertically), as illustrated in Figure 3. This provides vital information for training radar algorithms to identify pedestrians, versus the digital 4D form factor of say, a dog, crossing the road.

Figure 3. Advanced digital processing with radar scene emulation provides greater granularity of dynamic targets, such as a moving pedestrian.
The Road to Super Sensors Begins with Reliable Testing
From chip design to its fabrication and subsequent radar module testing, each step of the automotive radar design, development, and fabrication lifecycle demands rigorous testing.
“Automotive radar systems need more rigorous testing as they assume critical functions in many of today’s Currently, automakers and radar module providers test the functionality of their radar modules using both software and hardware. There are two key methods for hardware-based tests.”
There are many test challenges when working with mmWave frequencies for automotive radar applications. Engineers need to consider the test setup, ensure the test equipment can carry out ultra-wideband mmWave measurements, mitigate signal-to-noise ratio loss, and meet emerging standards requirements by different market regions for interference testing.
At the radar module level, testing of modern 4D and imaging radar modules requires test equipment that can provide more bandwidth and better distance accuracy.
Finally, the ultimate challenge is integrating the automotive radar into the ADAS and automated driving system and subjecting the algorithms from standard driving situations to the one-in-a-million corner case. The well-trained and tested radar super sensor system will ensure smoother and safer rides for passengers as more drivers take a back seat in the future.


Archive
- October 2024(44)
- September 2024(94)
- August 2024(100)
- July 2024(99)
- June 2024(126)
- May 2024(155)
- April 2024(123)
- March 2024(112)
- February 2024(109)
- January 2024(95)
- December 2023(56)
- November 2023(86)
- October 2023(97)
- September 2023(89)
- August 2023(101)
- July 2023(104)
- June 2023(113)
- May 2023(103)
- April 2023(93)
- March 2023(129)
- February 2023(77)
- January 2023(91)
- December 2022(90)
- November 2022(125)
- October 2022(117)
- September 2022(137)
- August 2022(119)
- July 2022(99)
- June 2022(128)
- May 2022(112)
- April 2022(108)
- March 2022(121)
- February 2022(93)
- January 2022(110)
- December 2021(92)
- November 2021(107)
- October 2021(101)
- September 2021(81)
- August 2021(74)
- July 2021(78)
- June 2021(92)
- May 2021(67)
- April 2021(79)
- March 2021(79)
- February 2021(58)
- January 2021(55)
- December 2020(56)
- November 2020(59)
- October 2020(78)
- September 2020(72)
- August 2020(64)
- July 2020(71)
- June 2020(74)
- May 2020(50)
- April 2020(71)
- March 2020(71)
- February 2020(58)
- January 2020(62)
- December 2019(57)
- November 2019(64)
- October 2019(25)
- September 2019(24)
- August 2019(14)
- July 2019(23)
- June 2019(54)
- May 2019(82)
- April 2019(76)
- March 2019(71)
- February 2019(67)
- January 2019(75)
- December 2018(44)
- November 2018(47)
- October 2018(74)
- September 2018(54)
- August 2018(61)
- July 2018(72)
- June 2018(62)
- May 2018(62)
- April 2018(73)
- March 2018(76)
- February 2018(8)
- January 2018(7)
- December 2017(6)
- November 2017(8)
- October 2017(3)
- September 2017(4)
- August 2017(4)
- July 2017(2)
- June 2017(5)
- May 2017(6)
- April 2017(11)
- March 2017(8)
- February 2017(16)
- January 2017(10)
- December 2016(12)
- November 2016(20)
- October 2016(7)
- September 2016(102)
- August 2016(168)
- July 2016(141)
- June 2016(149)
- May 2016(117)
- April 2016(59)
- March 2016(85)
- February 2016(153)
- December 2015(150)