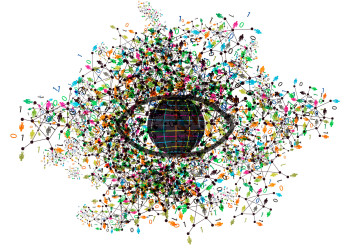
The pace of change in the Big Data space is fast and furious. From the technology side, the conversation has rapidly moved from Map Reduce to Spark. Driving innovations like this are progressive businesses adopting use cases involving streaming data and real time analytics.
Many of these companies have spent a lot of effort building the technical foundation necessary to obtain, process and analyze data. While success stories abound, there are many more companies struggling to partake in the “Big Data revolution.”
IT leaders often feel alone at the helm of Big Data strategy. When faced with this self-imposed responsibility, the outcomes tend to be ones few in the business appreciate. Such as well-understood use cases such as ETL offload, data warehouse cost optimization and storage.
Unfortunately, these efforts don’t really improve the top line, which is where executives now demand Big Data investments to impact. This is leading many companies toward a tipping point where they will either get it right or wrong with respect to realizing business value from Big Data Analytics.
Fact is, most executives in most companies cannot report the business value related to their Big Data efforts – looking to leverage existing and new data sources to answer and address tough questions about their business, competitors and markets. This represents a huge opportunity for leaders in many roles – IT, sales, marketing, supply chain, operations, and finance – to shift their Big Data efforts into high gear.
Technically, the challenge is to think beyond Big Data in terms of a “Do it Yourself” mode of stitching together a variety of open source and commercial technologies. Instead, imagine the possibilities of having access to cloud capabilities that support rapid exploration of business value oriented use cases.
Imagine as well the ability to have lines of business pay for these projects with their own opex budgets – giving them greater control but also much more “skin in the game” with respect to project success. If a use case employs internal data sources, and most use cases usually do, IT will be at the table to help.
Helping bridge the gap among IT and business is the emergence of the hybrid businessperson/analyst called the “Citizen Data Scientist,” who is data-oriented and desires better access to data and more helpful analysis tools. This person may not be a line of business executive or manager, or work in traditional IT.
The Citizen Data Scientist should be viewed as an exciting development for companies struggling to get more value from their investments in Big Data. They represent a key skillset that must be leveraged and understood within the context of the strategy a business employs around Big Data.
IT most certainly always plays a role, but without the support of a line of business sponsor to identify an opportunity or problem, as well as your Citizen Data Scientist, projects tend to fail. Reports of IT creating successful new analytics without business involvement early and often are few and far between. It’s collaboration among several individuals, at scale, that helps an organization “commercialize” analytics to the extent it can be as repeatable a process as any other in the business.
To reach that point, it’s helpful to view analytic use cases in a portfolio context – like a portfolio of financial instruments, each with different risk and reward profiles. These use cases can be rolled up to support a process a businessperson views as critical to their success.
A process like the consumer journey, from consideration to purchase and the post-purchase experience. There are numerous decisions within this journey that could be improved with access to new data sources and new forms of analytics. Collaboration among a business sponsor, an analyst or data science professional, and IT, can leverage a business process construct to identify a portfolio of use cases to prioritize and attack.
Prioritization happens along a number of dimensions:
• Business impact or value: how much can a decision or process be improved relative to the current state? What does it mean in hard currency?
• Data availability: what data sources are required and can they be accessed in a timely manner?
• Skills required: based on the desired outcome, what analytical skill will be required? What modeling type will be necessary? Do you need help from a third party to execute this?
• Timeline: how soon can the project be executed and operationalized?
If you are just starting off, focusing on analytics that support an existing decision can make more sense than one that implies re-engineering a process. A simple representation of these considerations serves as a clear method of communicating an actionable Big Data strategy to executives that scales. That is very important as these same executives start to wonder if Big Data hype can be realized in terms of tangible business value.
This article was originally published information-management.com and can be viewed in full here


Archive
- October 2024(44)
- September 2024(94)
- August 2024(100)
- July 2024(99)
- June 2024(126)
- May 2024(155)
- April 2024(123)
- March 2024(112)
- February 2024(109)
- January 2024(95)
- December 2023(56)
- November 2023(86)
- October 2023(97)
- September 2023(89)
- August 2023(101)
- July 2023(104)
- June 2023(113)
- May 2023(103)
- April 2023(93)
- March 2023(129)
- February 2023(77)
- January 2023(91)
- December 2022(90)
- November 2022(125)
- October 2022(117)
- September 2022(137)
- August 2022(119)
- July 2022(99)
- June 2022(128)
- May 2022(112)
- April 2022(108)
- March 2022(121)
- February 2022(93)
- January 2022(110)
- December 2021(92)
- November 2021(107)
- October 2021(101)
- September 2021(81)
- August 2021(74)
- July 2021(78)
- June 2021(92)
- May 2021(67)
- April 2021(79)
- March 2021(79)
- February 2021(58)
- January 2021(55)
- December 2020(56)
- November 2020(59)
- October 2020(78)
- September 2020(72)
- August 2020(64)
- July 2020(71)
- June 2020(74)
- May 2020(50)
- April 2020(71)
- March 2020(71)
- February 2020(58)
- January 2020(62)
- December 2019(57)
- November 2019(64)
- October 2019(25)
- September 2019(24)
- August 2019(14)
- July 2019(23)
- June 2019(54)
- May 2019(82)
- April 2019(76)
- March 2019(71)
- February 2019(67)
- January 2019(75)
- December 2018(44)
- November 2018(47)
- October 2018(74)
- September 2018(54)
- August 2018(61)
- July 2018(72)
- June 2018(62)
- May 2018(62)
- April 2018(73)
- March 2018(76)
- February 2018(8)
- January 2018(7)
- December 2017(6)
- November 2017(8)
- October 2017(3)
- September 2017(4)
- August 2017(4)
- July 2017(2)
- June 2017(5)
- May 2017(6)
- April 2017(11)
- March 2017(8)
- February 2017(16)
- January 2017(10)
- December 2016(12)
- November 2016(20)
- October 2016(7)
- September 2016(102)
- August 2016(168)
- July 2016(141)
- June 2016(149)
- May 2016(117)
- April 2016(59)
- March 2016(85)
- February 2016(153)
- December 2015(150)