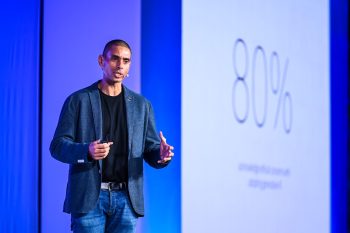
Written by: Bailey Martin, Tech Journalists, AOPG.
IBM Think, the tech and business conference that sets the stage for technology leaders, innovators, and industry experts from around the globe, is embarking on a world tour in 2024. As a hub for tech adoption and technological advancement in the ASEAN region, Singapore marks a key stop for IBM on August 15th. At this event, we can anticipate keynotes and presentations on IBM and its partners’ findings regarding AI adoption by enterprises, as well as AI readiness. We also eagerly await what IBM executives will share about their newest version of AI specialised for enterprises called ‘watsonx.’ Fortunately for Disruptive Tech News (DTN), ahead of the main event, IBM’s PR team had flown us out the day before to attend their media briefing and interview some of IBM’s experts.
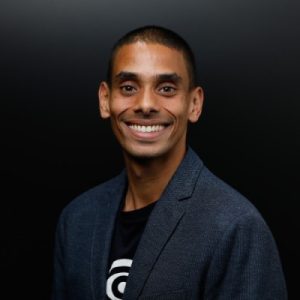
Nicholas Renotte, the Chief AI Engineer at IBM
From the list of such talented and tenured individuals, DTN jumped at the opportunity to interview Nicholas Renotte, the Chief AI Engineer at IBM. Nicholas Renotte is also a content creator with almost 300,000 subscribers on his YouTube channel, ‘Nicholas Renotte,’ where he shares key knowledge and expertise on navigating the challenges and configuration of Large Language Models (LLMs) and Generative AI (GenAI) models. In our interview with him, Nicholas shared his extensive knowledge and advice for all those who are looking to use open-source AI models such as watsonx. He also shared his insights extensively on the process of ‘fine tuning’ for AI and its advantages over AI (pre)training, especially for an open-source AI model such as IBM’s watsonx.
To any readers not familiar with this term, ‘fine-tuning’ is the process of customising or modifying a pre-trained model for specific tasks or purposes. It has gained prominence as a deep learning technique. Lastly, Nicholas also shares his thoughts on the phenomenon of AI hallucinations where missteps in data and pre-training lead to miscalculations and false analyses. With this in mind, let’s look at Nicholas’ answers to our questions in his own words.
1. What are the benefits to businesses that utilise the practice of fine-tuning over AI training from scratch?
“[Training AI from scratch] takes thousands of GPUs and thousands of hours to ensure that you get to a baseline. Some degree of fine-tuning typically happens after that. Where I think there’s a lot of opportunity for most organisations is looking at parameter-efficient fine-tuning. There are techniques like using low-rank adapters to effectively tag new weights onto a machine-learning model. You’ve also got techniques like ‘prompt-tuning’ or creating virtual tokens, which again can reduce the overhead when going and fine-tuning these LLMs…”
Nicholas continues, “Let’s say, for example, you just needed to go and perform or do some search based on your own content. There’s a way that you can go and do that using retrieval augmented generation, right? It’s effectively a fancy way of saying you take a chunk of your data or a number of chunks into your data, plug it into your LLM [and] you get an answer with that context. Fine-tuning is particularly useful when you need to use specific language on your LLM on specific language… you need to bake in a number of types of responses into that LLM.”
2. What are some of the pitfalls that could occur when using ‘finetuning’ techniques, which businesses and individuals should be aware of?
“I think one of the biggest things that we’ve seen is that there’s this phenomenon called catastrophic forgetting, where you have already gone and spent millions of dollars tuning or pre-training and then instruction tuning and then potentially fine-tuning begins to forget what it’s been trained on. You start to get this process of things which it was really good at [and now] not being so good anymore…”
About putting measures in place to offset these issues, Nicholas shared: “There are processes that you can implement or safeguard to protect yourself against this. For example, parameter-efficient fine-tuning helps avoid this issue because you’re effectively not changing your base weights; [instead,] you’re creating a new set of weights and training those, so you’ve effectively got a fallback that if that adapter doesn’t perform so well, you can just take that away and start again. I think appropriate checkpointing means that that you’re effectively saving stages when you’re going and fine-tuning one of these models, so you can go and backup…”
3. The world has seen numerous cases of AI ‘Hallucinations’. Is this simply down to flaws in the pre-training stages and development which could be avoided, or are AI hallucinations inevitable and something businesses should have contingencies in place for?
“[About machine learning models,] keep in mind that we’re trying to minimise the error, it’s almost impossible to eliminate it. So, hallucination to some degree is potentially likely to happen. There are ways to ensure that you hedge against this. One of the frameworks that we’ve started implementing with some of our clients is called the ‘Swiss Cheese framework’. When we go and deploy an LLM, we have our first ‘Swiss cheese layer’. Think of that as your input filtering. We make sure that we’re taking in the right prompts…”
“The next phase is where we have model layer guide rails. So, we make sure that we’re only passing through the data that we want… The third and final layer is probably the most important when it comes to hallucinations, and that’s when we go and generate an answer. Let’s say, for example, if we use RAG (Retrieval-Augmented Generation), we can return the context that is relevant to that specific question, right? We can calculate metrics, things like faithfulness and contextual accuracy grounded to determine whether or not the answer is relevant to the context. By taking a look at all of these metrics, we can determine whether or not, we’ve got a reasonably valid answer and a lot of the time if we don’t have all of those pieces in the right place or we don’t have the appropriate values for those metrics, that’s generally an estimate of potential hallucination…”
About IBM’s ‘watsonx Flows’, Nicholas explained: “You can literally set a flag, and you get hallucination metrics calculated which can then be rendered back to the user. The user can see when they might not necessarily have the right context to generate that response or when the response doesn’t necessarily match the context.”
4. What are some of the roadblocks or reservations around AI adoption in APAC? Does watsonx AI overcome any of these roadblocks?
“Yes, I think one of the really interesting things that I’m gonna talk about at my keynote tomorrow is that we’ve got 45% of organisations now experimenting with GenAI… But only 10% of those are getting into production… When we go and start up a GenAI project, we’ve got a ton of stakeholders. We need a prompt engineer to go and write prompts. We need a data scientist to go and validate experiments. We need someone to handle a database. We need a line of businesspersons to go and test and validate and make sure that you’ve got the right prompts being generated [&] that it’s going to generate value. Every team is fundamentally going to want to do something slightly different. Maybe they want to use a different LLM, maybe they want to use different tools. Maybe one team codes in JavaScript, and one codes in Python. So, you’ve now got like this disconnect between all of these teams at one GenAI and they want it now, but they all do it a little bit differently…”
Nicholas explains how watsonx can be used to deal with this issue, saying: “…One of the ways that, that we sort of handle this is like with watsonx, right? Let’s say, for example, everyone wanted to use a different LLM you’ve got the ability to do that. It’s like a multimodal approach. You can use Meta Llama if you want to, you can use IBM’s models if you want to, you can go and use something else, you can even bring your own model if you want to.”
5. Is there anything important you’d like to share with our readers on watsonx AI?
“I think the most important thing is to start taking a look at how you’re going to get these LLMs out into production or these capabilities out into production. [Businesses] started experimenting a lot, but you truly need to go and see value when it comes to leveraging these capabilities…”
Nicholas continues “If you start thinking about how you’re going to finish [meaning utilise machine learning models into production], it helps inform how you start and where you need to go to start. So, do you need an API does it need to be embedded somewhere? Do you need specific regulations around this because it’s touching a particular use case? So think about where you want to end before you just start kicking off with LLMs because there’s a lot of stuff out there and not all of it is particularly suited for business.”
The Path Forward for LLMs and Generative AI with IBM
Thanks to Nicholas Renotte’s insights from this interview, we now have a clearer understanding of the essential precautions and recommended strategies for effectively utilising LLMs and GenAI models. With watsonx solutions being open-sourced, IBM leaves room for innovation by all who use it and makes it easier for organisations to fine-tune their model to their specific requirements. There are also methods to have layered checkpoints and frameworks to reduce the instances of potential AI hallucinations, although they will most likely remain a hazard to keep on the lookout for. A big thank you to Nicholas Renotte for sharing his expertise with us!


Archive
- October 2024(44)
- September 2024(94)
- August 2024(100)
- July 2024(99)
- June 2024(126)
- May 2024(155)
- April 2024(123)
- March 2024(112)
- February 2024(109)
- January 2024(95)
- December 2023(56)
- November 2023(86)
- October 2023(97)
- September 2023(89)
- August 2023(101)
- July 2023(104)
- June 2023(113)
- May 2023(103)
- April 2023(93)
- March 2023(129)
- February 2023(77)
- January 2023(91)
- December 2022(90)
- November 2022(125)
- October 2022(117)
- September 2022(137)
- August 2022(119)
- July 2022(99)
- June 2022(128)
- May 2022(112)
- April 2022(108)
- March 2022(121)
- February 2022(93)
- January 2022(110)
- December 2021(92)
- November 2021(107)
- October 2021(101)
- September 2021(81)
- August 2021(74)
- July 2021(78)
- June 2021(92)
- May 2021(67)
- April 2021(79)
- March 2021(79)
- February 2021(58)
- January 2021(55)
- December 2020(56)
- November 2020(59)
- October 2020(78)
- September 2020(72)
- August 2020(64)
- July 2020(71)
- June 2020(74)
- May 2020(50)
- April 2020(71)
- March 2020(71)
- February 2020(58)
- January 2020(62)
- December 2019(57)
- November 2019(64)
- October 2019(25)
- September 2019(24)
- August 2019(14)
- July 2019(23)
- June 2019(54)
- May 2019(82)
- April 2019(76)
- March 2019(71)
- February 2019(67)
- January 2019(75)
- December 2018(44)
- November 2018(47)
- October 2018(74)
- September 2018(54)
- August 2018(61)
- July 2018(72)
- June 2018(62)
- May 2018(62)
- April 2018(73)
- March 2018(76)
- February 2018(8)
- January 2018(7)
- December 2017(6)
- November 2017(8)
- October 2017(3)
- September 2017(4)
- August 2017(4)
- July 2017(2)
- June 2017(5)
- May 2017(6)
- April 2017(11)
- March 2017(8)
- February 2017(16)
- January 2017(10)
- December 2016(12)
- November 2016(20)
- October 2016(7)
- September 2016(102)
- August 2016(168)
- July 2016(141)
- June 2016(149)
- May 2016(117)
- April 2016(59)
- March 2016(85)
- February 2016(153)
- December 2015(150)