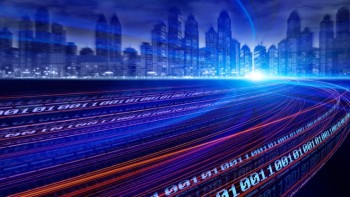
Predictive analytics is the practical result of Big Data and business intelligence (BI). What do you do when your business collects staggering volumes of new data? Today’s business applications are raking in mountains of new customer, market, social listening, and real-time app, cloud, or product performance data. Predictive analytics is one way to leverage all of that information, gain tangible new insights, and stay ahead of the competition.
Organizations use predictive analytics in a variety of different ways, from predictive marketing and data mining to applying machine learning (ML) and artificial intelligence (AI) algorithms to optimize business processes and uncover new statistical patterns. It’s basically computers learning from past behavior about how to do certain business processes better and deliver new insights into how your organization really functions. But before we get into all of the fascinating ways businesses and technology companies are employing predictive analytics to save time, save money, and gain an edge over the rest of the market, it’s important to talk about exactly what predictive analytics is and what it’s not.
What Is Predictive Analytics?
Predictive analytics isn’t a black-and-white concept or a discrete feature of modern database managers. It’s a bunch of data analysis technologies and statistical techniques rolled up under one banner. The core technique is regression analysis, which predicts the related values of multiple, correlated variables based on proving or disproving a particular assumption. Predictive analytics is about recognizing patterns in data to project probability, according to Allison Snow, Senior Analyst of B2B Marketing at Forrester.
“It’s key to recognize that analytics is about probabilities, not absolutes,” explained Snow, who covers the predictive marketing space. “Unlike traditional analytics, when applying predictive analytics, one doesn’t know in advance what data is important. Predictive analytics determine what data is predictive of the outcome you wish to predict.”
Think about a sales representative looking at a lead profile in a customer relationship management (CRM) platform such as Salesforce.com$25.00 at Salesforce.com. Let’s say the assumption is, the lead will buy your product. Other assumptions are that the variables are product cost, the lead’s role within a business, and the company’s current profitability ratio. Now plop those variables into a regression equation and voila! You’ve got a predictive model from which to extrapolate an effective strategy for pitching and selling a product to the right leads.
Aside from regression analysis (the intricacies and subsets of which you can read more about in this Harvard Business Review primer), predictive analytics is also using progressively more data mining and ML. Data mining is exactly what it sounds like: you examine large data sets to discover patterns and uncover new information. ML techniques are, with greater regularity, becoming the sifting pans and pickaxes for finding the gold data nuggets. ML innovations such as neural networks and deep learning algorithms can process these unstructured data sets faster than a traditional data scientist or researcher, and with greater and greater accuracy as the algorithms learn and improve. It’s the same way IBM Watson works, and open-source toolkits such as Google’s TensorFlow and Microsoft’s CNTK offer ML functionality along the same lines.
The big change feeding into the predictive analytics boom is not just the advancement of ML and AI, but that it’s not just data scientists using these techniques anymore. BI and data visualization tools, along with open-source organizations like the Apache Software Foundation, are making Big Data analysis tools more accessible, more efficient, and easier to use than ever before. ML and data analysis tools are now self-service and in the hands of everyday business users—from our salesperson analyzing lead data or the executive trying to decipher market trends in the boardroom to the customer service rep researching common customer pain points and the social media marketing manager gauging follower demographics and social trends to reach the right targeted audience with a campaign. These use cases are just the tip of the iceberg in exploring all of the ways predictive analytics is changing business, many more of which we’ll get into below.
That said, predictive analytics is not like a crystal ball or Biff Tannen’s sports almanac from Back to the Future 2. The algorithms and models can’t tell your business beyond the shadow of a doubt that its next product will be a billion-dollar winner or that the market is about to tank. Data is still a means to make an educated guess; we’re simply a lot better educated than we used to be.
Breaking Down Predictive, Prescriptive, and Descriptive Analytics
In another Forrester report entitled ‘Predictive Analytics Can Infuse Your Applications With An ‘Unfair Advantage,'” Principal Analyst Mike Gualtieri points out that “the word ‘analytics’ in ‘predictive analytics’ is a bit of a misnomer. Predictive analytics is not a branch of traditional analytics such as reporting or statistical analysis. It is about finding predictive models that firms can use to predict future business outcomes and/or customer behavior.”
In short, Snow explained that the term “predictive” inherently denotes likelihood over certainty, breaking down the analytics tooling landscape and how it factors into prescriptive analytics.
“Descriptive analytics, while not particularly ‘advanced,’ simply capture things that happened,” said Snow. “Descriptive or historical analytics is the foundation on which an algorithm might be developed. These are simple metrics but often too voluminous to manage without an analytics tool.
“Generally speaking, dashboards and reporting are the most common use for predictive analytics within organizations today. These tools often lack the link to business decisions, process optimization, customer experience, or any other action. In other words, models produce insights but not explicit instructions on what to do with them. Prescriptive analytics is where insight meets action. They answer the question, ‘I now know the probability of an outcome [and] what can be done to influence it in the direction that’s positive for me,’ whether that be preventing customer churn or making a sale more likely.”
Predictive Analytics Is Everywhere
As the BI landscape evolves, predictive analytics is finding its way into more and more business use cases. Tools such as our Editors’ Choices Tableau Desktop $999.00 at Tableau Software and Microsoft Power BI Free at Microsoft sport intuitive design and usability, and large collections of data connectors and visualizations to make sense of the massive volumes of data businesses import from sources such as Amazon Elastic MapReduce (EMR), Google BigQuery, and Hadoop distributions from players such as Cloudera, Hortonworks, and MapR. These self-service tools don’t necessarily have the most advanced predictive analytics features yet, but they make the Big Data a lot smaller and easier to analyze and understand.
Snow said there is a broad series of use cases for predictive analytics in business today, from detecting point-of-sale (POS) fraud, automatically adjusting digital content based on user context to drive conversions, or initiating proactive customer service for at-risk revenue sources. In B2B marketing, Snow said enterprises and SMBs use predictive marketing for the same reasons they use any strategy, tactic, or technology: to win, retain, and serve customers better than those that don’t.
Drilling down deeper, Snow identified three categories of B2B marketing use cases she said dominate early predictive success and lay the foundation for more complex use of predictive marketing analytics.
1. Predictive Scoring: Prioritizing known prospects, leads, and accounts based on their likelihood to take action.
“The most common entry point for B2B marketers into predictive marketing, predictive scoring adds a scientific, mathematical dimension to conventional prioritization that relies on speculation, experimentation, and iteration to derive criteria and weightings,” said Snow. “This use case help sales and marketers identify productive accounts faster, spend less time on accounts less likely to convert, and initiate targeted cross-sell or upsell campaigns.”
2. Identification Models: Identifying and acquiring prospects with attributes similar to existing customers.
“In this use case, accounts that exhibited desired behavior (made a purchase, renewed a contract, or purchased additional products and services) serve as the basis of an identification model,” said Snow. “This use case help sales and marketers find valuable prospects earlier in the sales cycle, uncover new marketers, prioritize existing accounts for expansion, and power account-based marketing (ABM) initiatives by bringing to the surface accounts that can reasonably be expected to be more receptive to sales and marketing messages.”
3. Automated Segmentation: Segment leads for personalized messaging.
“B2B marketers have traditionally been able to segment only by generic attributes, like industry, and did so with such manual effort that personalization applied only to highly prioritized campaigns,” said Snow. “Now, attributes used to feed predictive algorithms can now be appended to account records to support both intricate and automated segmentation. This use case help sales and marketers drive outbound communications with relevant messages, enable substantial conversations between sales and prospects, and inform content strategy more intelligently.”
BI tools and open-source frameworks such as Hadoop are democratizing data as a whole but, aside from B2B marketing, predictive analytics is also being baked into more and more cloud-based software platforms across a host of industries. Take online dating company eHarmony’s Elevated Careers website and the handful of other vendors in the “predictive analytics for hiring” space. These platforms are still very much in their early days, but the idea of using data to predict which job seekers are the best fit for specifics jobs and companies has the potential to reinvent how human resources (HR) managers recruit talent.
Help desk providers such as Zendesk $5.00 at Zendesk have also begun adding predictive analytics capabilities to help desk software. The company imbued its platform with predictive powers to help customer service reps spot problem areas with a data-driven early warning system called Satisfaction Prediction. The feature uses a ML algorithm to process satisfaction survey results, throwing variables including time to resolve a ticket, customer service response latency, and specific ticket wording into a regression algorithm to calculate a customer’s projected satisfaction rating.
We’re also seeing predictive analytics make a big impact to the bottom line on industrial scale and with the Internet of Things (IoT). Google uses ML algorithms in its data centers to run predictive maintenance on the server farms powering itsGoogle Cloud Platform$6,092.00 at Google (GCP) public cloud infrastructure. The algorithms use data on weather, load, and other variables to adjust data center cooling pumps preemptively and significantly reduce power consumption.
This kind of predictive maintenance is becoming commonplace in factories as well. Enterprise tech companies such as SAP offer predictive maintenance and service platforms using sensor data from connected IoT manufacturing devices to predict when a machine is at risk for mechanical problems or failure. Tech companies such as Microsoft are also exploring predictive maintenance for aerospace apps, putting Cortana to work on analyzing sensor data from aircraft engines and components.
The list of potential business apps goes on and on, from how predictive analytics is changing the retail industry to fintech start-ups using predictive modeling on fraud analysis and financial transaction risk. We’ve only scratched the surface, both in the ways different industries could integrate this type of data analysis and the depths to which predictive analytics tools and techniques will redefine how we do business in concert with the evolution of AI. As we inch closer to truly mapping an artificial brain, the possibilities are endless.
This article was originally published on www.pcmag.com and can be viewed in full


Archive
- October 2024(44)
- September 2024(94)
- August 2024(100)
- July 2024(99)
- June 2024(126)
- May 2024(155)
- April 2024(123)
- March 2024(112)
- February 2024(109)
- January 2024(95)
- December 2023(56)
- November 2023(86)
- October 2023(97)
- September 2023(89)
- August 2023(101)
- July 2023(104)
- June 2023(113)
- May 2023(103)
- April 2023(93)
- March 2023(129)
- February 2023(77)
- January 2023(91)
- December 2022(90)
- November 2022(125)
- October 2022(117)
- September 2022(137)
- August 2022(119)
- July 2022(99)
- June 2022(128)
- May 2022(112)
- April 2022(108)
- March 2022(121)
- February 2022(93)
- January 2022(110)
- December 2021(92)
- November 2021(107)
- October 2021(101)
- September 2021(81)
- August 2021(74)
- July 2021(78)
- June 2021(92)
- May 2021(67)
- April 2021(79)
- March 2021(79)
- February 2021(58)
- January 2021(55)
- December 2020(56)
- November 2020(59)
- October 2020(78)
- September 2020(72)
- August 2020(64)
- July 2020(71)
- June 2020(74)
- May 2020(50)
- April 2020(71)
- March 2020(71)
- February 2020(58)
- January 2020(62)
- December 2019(57)
- November 2019(64)
- October 2019(25)
- September 2019(24)
- August 2019(14)
- July 2019(23)
- June 2019(54)
- May 2019(82)
- April 2019(76)
- March 2019(71)
- February 2019(67)
- January 2019(75)
- December 2018(44)
- November 2018(47)
- October 2018(74)
- September 2018(54)
- August 2018(61)
- July 2018(72)
- June 2018(62)
- May 2018(62)
- April 2018(73)
- March 2018(76)
- February 2018(8)
- January 2018(7)
- December 2017(6)
- November 2017(8)
- October 2017(3)
- September 2017(4)
- August 2017(4)
- July 2017(2)
- June 2017(5)
- May 2017(6)
- April 2017(11)
- March 2017(8)
- February 2017(16)
- January 2017(10)
- December 2016(12)
- November 2016(20)
- October 2016(7)
- September 2016(102)
- August 2016(168)
- July 2016(141)
- June 2016(149)
- May 2016(117)
- April 2016(59)
- March 2016(85)
- February 2016(153)
- December 2015(150)