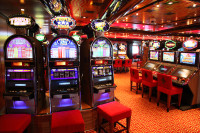
It’s estimated that 3 to 5 percent of people who gamble develop an addiction to the activity, which can lead to an array of problems for gamblers, their families, and society at large. Millions are spent annually treating people with gambling addiction, but now some gaming institutions are exploring innovative ways of identifying problem gamblers in real-time through an innovative application of machine learning.
Gambling is a big business, bringing in $500 billion in revenues around the world every year. Thanks to the rise of Internet gambling and the popularity of fantasy sports games like FanDuel and DraftKings, the business model appears to be on solid footing for years of growth.
But as it grows, the industry must grapple with the problem of gambling addiction, which cuts across all social and demographic groups and can impact anybody. Currently, a bright light is being shone on the problem in the UK, where regulated gambling has been legal for decades, and where casinos and betting parlors are easily accessed.
According to BetBuddy CEO Simo Dragicevic, over half of the UK population gambles on a regular basis, putting them at risk of developing into problem gamblers, for whom the anticipation and thrill of gambling creates a natural high that can become addictive. The UK government is stepping up to address the problem, and doctors are even prescribing drugs like Naltrexone, which is normally used to treat drug addicts and alcoholics, in an effort to stave off the craving of gamblers.
BetBuddy, which is based in the UK, develops a software product called PowerCrunch that uses data mining and machine learning techniques to detect high-risk or problem gambling while the player is still engaged in the activity. The software, which was built using machine learning automation software from H2O, works by calculating a risk score for each gambler based on his or her actions, and then automatically sending out personalized and targeted messages and tests to the players, thereby helping to understand their own behavior and its implications, and possibly steering them away from potential problems.
Detecting Problem Gamblers
A lot of research has gone into the BetBuddy system, which is based on a three-tier model of gambling-related data that BetBuddy researchers published in the Journal of Gambling Studies in 2013.
According to BetBuddy, the first tier analyzes exhibited behavior, or data collected directly from casino management system. The second tier looks at declared behavior, or the results of self-assessment test. The third tier revolves around inferred behavior, which is deduced by combining results from the first two to create a predictive model based on actual data gleaned from problem gamblers (anonymized to protect privacy).
Two years ago, BetBuddy set out to improve the accuracy of its inferred behavior model by working with the Machine Learning Group from City University London. The collaboration was funded by the UK’s innovation agency, Innovate UK, and involved contributions from the Engineering and Physical Sciences Research Council (EPSRC) and the Defense Science and Technology Laboratory (Dstl).
The challenge that BetBuddy faced was not trivial. While the vast majority of people who gamble do not become addicted to it, history shows that a small percentage will succumb to the gambling’s dark side. The key to success for BetBuddy lies in its ability to accurately identify the complex behavioral pathways that lead to the onset of gambling addiction, and then build a predictive software program that signals when those pathways are being filled using a combination of artificial neural networks, random forests, Bayesian network, and logistic regression algorithms.
The research yielded some interesting results–namely that using random forest algorithms could elevate the accuracy of BetBuddy’s forecasts of problem gamblers to 87 percent. That compares favorably to earlier peer-reviewed studies using a Harvard Medical School data set and methodology, which produced accuracies in the 62 to 67 percent accuracy range.
The key driver to the increased performance, the company says, was the technique that BetBuddy used to detect changes in behavior of players as they approach the point at which they experience harm.
Putting ML Into Production
The next challenge BetBuddy faced was operationalizing the insights generated by its platform, and that’s where the machine learning platform from H2O comes into the picture. According to H2O, BetBuddy analyzed a range of machine learning platforms that are compatible with Java, including Spark, Weka, and Mahout, but eventually settled on H2O.
BetBuddy’s selection of H2O hinged on three main factors, Dragicevic says. First, the H2O software is not tightly tied to Hadoop, which BetBuddy wanted to avoid. It also doesn’t have the “experimental” tag that other platform shave, and doesn’t suffer from open source licensing restrictions (although H2O’s software is open source), the BetBuddy CEO says.
H2O also helped BetBuddy operationalize the machine learning models into PowerCrunch, which was written in Java. Because H2O could expose the models as POJOs—or plain old Java objects—it simplified the process of putting the machine learning models into action.
“The only platform that scored highly on all of BetBuddy’s selection criteria was H2O,” Dragicevic says. “These considerations, coupled with strategic developments undertaken by H2O to integrate with the most promising platforms in the market, such as Spark, gave BetBuddy confidence in its choice.”
Real World Impact
PowerCrunch is still fairly new, but BetBuddy’s “responsible gambling” solution is showing signs of adoption. In particular, the Ontario Lottery and Gaming Corporation (OLG) tested the software and concluded that nine out of 10 players found the software helpful in managing their personal gambling.
Going forward, BetBuddy and City University are largely focused on opening up the underlying algorithms used in the system. Regulators and gaming executives, in particular, are interested in how BetBuddy’s software makes its predictions. The company is utilizing H2O’s model training analysis functionality in the hope of adding more transparency to the random forest and neural network algorithms that underlie the program.
As the gambling industry grows, casinos have a responsibility to identify the minority of gamblers who can’t rein themselves in. Innovative applications of data science, such as the one that BetBuddy is developing, show that responsibility in the gambling hall doesn’t have to come at the cost of excluding 95 percent of the population from having a little fun now and then.
This article was originally published datanami.com and can be viewed in full here


Archive
- October 2024(44)
- September 2024(94)
- August 2024(100)
- July 2024(99)
- June 2024(126)
- May 2024(155)
- April 2024(123)
- March 2024(112)
- February 2024(109)
- January 2024(95)
- December 2023(56)
- November 2023(86)
- October 2023(97)
- September 2023(89)
- August 2023(101)
- July 2023(104)
- June 2023(113)
- May 2023(103)
- April 2023(93)
- March 2023(129)
- February 2023(77)
- January 2023(91)
- December 2022(90)
- November 2022(125)
- October 2022(117)
- September 2022(137)
- August 2022(119)
- July 2022(99)
- June 2022(128)
- May 2022(112)
- April 2022(108)
- March 2022(121)
- February 2022(93)
- January 2022(110)
- December 2021(92)
- November 2021(107)
- October 2021(101)
- September 2021(81)
- August 2021(74)
- July 2021(78)
- June 2021(92)
- May 2021(67)
- April 2021(79)
- March 2021(79)
- February 2021(58)
- January 2021(55)
- December 2020(56)
- November 2020(59)
- October 2020(78)
- September 2020(72)
- August 2020(64)
- July 2020(71)
- June 2020(74)
- May 2020(50)
- April 2020(71)
- March 2020(71)
- February 2020(58)
- January 2020(62)
- December 2019(57)
- November 2019(64)
- October 2019(25)
- September 2019(24)
- August 2019(14)
- July 2019(23)
- June 2019(54)
- May 2019(82)
- April 2019(76)
- March 2019(71)
- February 2019(67)
- January 2019(75)
- December 2018(44)
- November 2018(47)
- October 2018(74)
- September 2018(54)
- August 2018(61)
- July 2018(72)
- June 2018(62)
- May 2018(62)
- April 2018(73)
- March 2018(76)
- February 2018(8)
- January 2018(7)
- December 2017(6)
- November 2017(8)
- October 2017(3)
- September 2017(4)
- August 2017(4)
- July 2017(2)
- June 2017(5)
- May 2017(6)
- April 2017(11)
- March 2017(8)
- February 2017(16)
- January 2017(10)
- December 2016(12)
- November 2016(20)
- October 2016(7)
- September 2016(102)
- August 2016(168)
- July 2016(141)
- June 2016(149)
- May 2016(117)
- April 2016(59)
- March 2016(85)
- February 2016(153)
- December 2015(150)